Automating Inequality: How High-Tech Tools Profile, Police, and Punish the Poor
Virginia Eubanks
2018, 272 pp, St. Martin’s Press
What if we thought differently about how to integrate human and machine agencies?
As I sat down in to write this review of Virginia Eubanks’ latest book, Automating Inequality: How High-Tech Tools Profile, Police, and Punish the Poor, I couldn’t help but consider it in light of the growing restiveness among tech workers in response to their companies’ perceived ethical lapses. Rank and file employees have begun to speak out against the use of big data-driven software systems and infrastructure for ethically questionable ends like warfare, policing, and family separation at the United States-Mexico border. To date, these protests have mired several public-private contracts between government agencies and some of the world’s biggest tech companies in controversy, including Google’s Project Maven, a collaboration with the Pentagon to target drone strikes; Microsoft’s Azure, a cloud infrastructure for Immigration and Customs Enforcement to target protesters of family and child detention; and Amazon’s surveillance system Rekognition, sold to Florida police departments to track suspected and potential criminals in public spaces.
Against this background, Automating Inequality is an urgently needed account of the ethical risks of automated, data-driven decision making. This book focuses on its impact on the most vulnerable people in our societies, but these cases should also be understood as bellwethers for how automation is becoming integrated into the lives of everyone in the United States.
Automating Inequality raises serious questions about what is to become of human agency in a digitally automated world. It provides timely case studies for tech workers, policy makers, and anyone seeking to understand the social impacts of computing technologies and reevaluate the ethical frameworks that structure digital innovation and, ultimately, the changing landscape in which all of us access resources, care for each other, and build our lives.
Building the Digital Poorhouse
Eubanks offers detailed accounts of how the automation of public benefits decisions in Los Angeles, Pennsylvania’s Allegheny County, and the state of Indiana prevents poor people from accessing the services they need. These applications of computing technologies create what she calls “the digital poorhouse.” In this modern version of the Dickensian workhouse, digital surveillance, information sharing between social services and criminal justice agencies, and automated punishment for minor infractions limit the life chances of the poor and their children just as certainly as physical confinement.
The chapter “Automating Eligibility in the Heartland” opens with the story of Sophie Stipes. At the age of 6, Sophie had a number of health problems that require a gastric tube and special formula to ensure proper nutrition and expensive diapers. The cost of her care was as high as $6,000 a month. Benefits supporting the cost of her care were automatically withheld in 2008 following the roll out of a joint IBM/Affiliated Computer Services benefits automation system because her mother failed to sign a new form—she had received no notification that it was necessary. In this case, the replacement of human caseworkers and a traditional face-to-face service model with digital automation produced new vulnerabilities amongst citizens already facing significant hardships.
Critically, Eubanks shows that Sophie’s tragedy wasn’t a simple administrative oversight or computer slip up. The automated system was calibrated by its human designers to default to reducing benefits whenever possible. The assumption that a missing data point—absence of a form for Sophie—represents a willful lack of “compliance” and triggers a withdrawal of benefits was a design choice based on that overall goal of benefits reduction. Eubanks argues that while the decision-making apparatus may be newly computerized, the decisions it makes are directed by the centuries-old belief that the poor are untrustworthy—that “they are sneaky and prone to fraudulent claims, and their burdensome use of public resources must be repeatedly discouraged” (81).
Eubanks also examines the Allegheny Family Screening Tool, which predicts which children may be in need of intervention by social services agencies. The system indicates the risk of abuse or neglect a child faces using a single number, on a scale from 1 to 20. This number is produced by combing through large sets of school, criminal justice, health, and family service records from the child’s entire life, as well as from the lives of their parents and relatives, and aggregating them in a multi-agency county database. In most cases, social service caseworkers then use this number to determine whether to intervene on reports of child abuse and neglect. For scores of 20, the system automatically initiates an investigation.
The Allegheny tool uses past events—even events during the childhood of a child’s parent or grandparent—to determine future surveillance and intervention for families in the system. Eubanks argues that this system unfairly ties poor people to their pasts and brings the misfortunes and missteps of a child’s older relatives to bear on their life chances. Moreover, Eubanks shows that the data this system is based on is itself biased, reflecting racist attitudes towards the parenting practices of African Americans and classist judgments about parenting that make the exigencies of poverty look like abuse.
Rather than making judgments about the risk of abuse fairer, the system makes the decision trees programmed in by human designers even more rigid and permanent. It reduces the ability of human workers to make choices and the ability of clients to submit appeals that are reviewed in a complete and timely fashion. It reduces people’s ability to seek corrections to a child’s or adult’s record; for example, to remove nuisance complaints submitted without any evidence of abuse. One fascinating part of this chapter is Eubanks’s analysis of the ways that the Allegheny tool shifted the decision-making practices of case workers even when they were allowed to make judgements about referring cases to investigation.
Ultimately, Eubanks demonstrates how algorithmic decision-making aids make it harder for individuals to change and grow, further entrenching patterns of intergenerational poverty and targeting poor people for intensified surveillance over the course of their lifetimes—and their children’s, and even their children’s children.
Imagining Automation Otherwise
But could it be otherwise? My disciplinary roots in feminist science studies and my current work in user research, innovation, and speculative futures, prime me to pursue this question. Recent work and teaching among tech workers and engineering students also has helped me consider where small changes could have the biggest impacts.
We might ask ourselves, our colleagues, and our organizations, What decisions is it appropriate for computers to make by default? If we do turn decision making over to machines, how should we calibrate the default options? Does the algorithmic automation of the delivery of services have to default to withholding services from eligible people and people in need? What if the default setting was to approve cases instead? Should the algorithmic identification of children at risk of abuse or neglect ever be turned into a single number? And should computers be entrusted with automating the escalation of any child abuse cases?
We can also ask, What if we thought differently about how to integrate human and machine agencies? What if human input was treated as part of the necessary infrastructure for making decisions that will intimately shape the life chances and everyday experiences of our fellow citizens? For example, building slowness into systems is gaining traction as a way to curb the likely ethical abuses of automated decision making around health, food, and shelter. What if we built in moments of pause when specialized human staff with advanced ethical, sociocultural, legal, and technical training could evaluate computer automated decisions?
We should also consider how to create disjunctures between surveillance and prediction, and prediction and enforcement, to make room for human agency. These systems might be less efficient, but there is a growing consensus that we need better guardrails around the ability of automated systems to determine how we humans live our lives.
As Automating Inequality demonstrates, technology companies are given incredible power to define the rules of society and to enforce the standards by which we live. While political and economic pressure to reduce costs and increase speed certainly exist, systems builders already budget in time and money for slow processes like maintenance and repair. Ethically sophisticated human decision makers could similarly be treated as a feature of data-driven decision-making systems, not as a bug.
There also needs to be greater oversight of the systems builders who set the defaults for automated systems based on their professional training and judgment. Whether we like it or not, human agency is already built into automated, computerized systems. When designers set defaults, program in a particular set of options, build a menu for program operators, and connect surveillance to prediction to enforcement in particular ways, they are building their agency into the machine. Despite this enormous influence, their roles, their qualifications for making decisions that will affect the private lives of thousands or millions of ordinary people, and their assumptions about “human nature” mostly remain a mystery once the system is deployed. Human agency is everywhere in automated systems, but it is built in haphazardly. As a result, the automated systems we have today create more suffering and social problems than they solve.
So how could algorithmic decision making be done otherwise? Using technology to create better worlds starts by recognizing that human agencies, based on particular humans’ values, are built into every automated system designed to date. Since these systems are made to scale to large numbers of people, the designers of these systems have a special responsibility to develop a robust sociotechnical imagination.
Eubanks takes pains to show that, in some cases, damaging systems are designed by good people with the best of intentions. But Automating Inequality is a testament to the fact that intentions are not enough. Systems designers must understand how technology has shaped the lives of people and whole societies in the past. They must also have the contextual background and critical capacity to imagine the wide array of impacts each new tool will have on societies in the future. And the bad must be given as much weight as the good in these scenarios.
Ultimately, a society-wide effort is required to deliberate and draw boundaries around how we want to use algorithmic decision-making tools, potentially including changes to legislation, new legal cases in the courts, changes to professional training of engineers and business school students, changes in hiring and promotion policies within tech companies, the elevation of people with new kinds of social expertise in those companies, and more.
But the first step toward finding ways to live well with machines is recognizing that they are ours, they act based on the values we give them, and we have the power to decide how we want them to shape our lives.
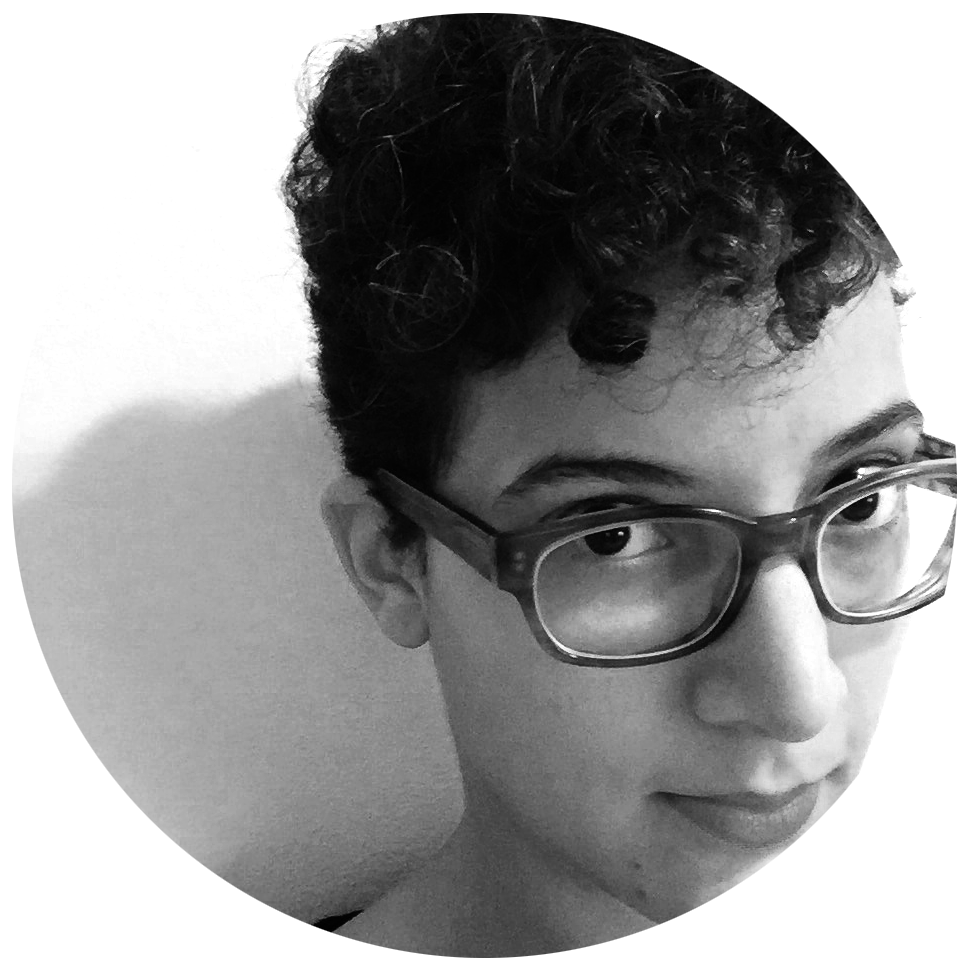
Related Articles
Agency is our EPIC2019 conference theme —Read more!
Rethinking Financial Literacy with Design Anthropology, Marijke Rijsberman
Shining a Light on Agency, Emma Rose & Robert Racadio
Gaming Evidence: Power, Storytelling and the “Colonial Moment” in a Chicago Systems Change Project, Nathan Heintz
0 Comments