This paper proposes a model of researcher-AI interaction and argues that ethnographers can smartly leverage AI in...
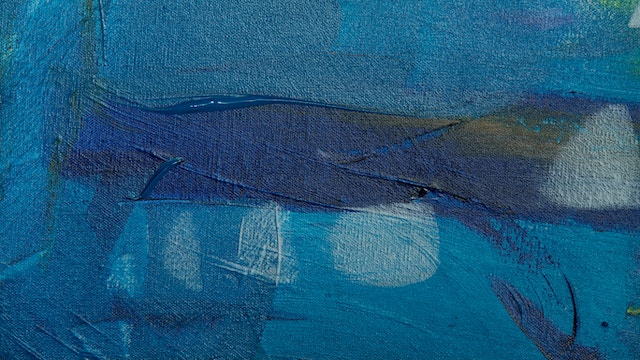
This paper proposes a model of researcher-AI interaction and argues that ethnographers can smartly leverage AI in...
This paper provides a theoretical alternative to the prevailing perception of machine learning as synonymous with...
A design executive who has led several multidisciplinary teams and organizations at global companies such as...
Learn models and principles to ensure organizations are creating, using, and deploying AI that coworkers, customers, and society can trust. Overview Our lives are directed, enriched, influenced, and sometimes...
The not-too-distant future may bring more ubiquitous personal computing technologies seamlessly integrated into people's lives, with the potential to augment reality and support human cognition. For such technology to be truly assistive to people, it must be context-aware. Human experience of...
A key challenge in carrying out product design research is obtaining rich contextual information about use in the wild. We present a method that algorithmically mediates between participants, researchers, and objects in order to enable real-time collaborative sensemaking. It facilitates contextual...
Case Study—We consider new expectations for ethnographic observation and sensemaking in the next 20-25 years, as technology industry ethnographers' work unfolds in the increasing presence of the type of analytical capabilities specially trained (and self-training) machines can do ‘better’ and...
The successes of technology companies that rely on data to drive their business hints at the potential of data science and machine learning (DS/ML) to reshape the corporate world. However, despite the headway made by a few notable titans (e.g., Google, Amazon, Apple) and upstarts, the advances...
The wide-spread deployment of machine learning tools within healthcare is on the horizon. However, the hype around “AI” tends to divert attention toward the spectacular, and away from the more mundane and ground-level aspects of new technologies that shape technological adoption and integration....
PechaKucha Presentation When we study human systems and organizations we have a job that requires to empathize or at the very least be compassionate towards the experiences others are having. This allows to understand their goals, problems, and how we can best make their lives better. When...