This paper provides a theoretical alternative to the prevailing perception of machine learning as synonymous with speed and efficiency. Inspired by ethnographic fieldwork and grounded in pragmatist philosophy, we introduce the concept of “data friction” as the situation when encounters between held beliefs and data patterns posses the potential to stimulate innovative thinking. Contrary to the conventional connotations of “speed” and “control,” we argue that computational methods can generate a productive dissonance, thereby fostering slower and more reflective practices within organizations. Drawing on a decade of experience in participatory data design and data sprints, we present a typology of data frictions and outline three ways in which algorithmic techniques within data science can be reimagined as “friction machines”. We illustrate these theoretical points through a dive into three case studies conducted with applied anthropologist in the movie industry, urban planning, and research.
Introduction
One of the most prevalent imaginaries around machine learning is that it supports speed and efficiency (Kitchin, 2014a). From doctors being able to spot early signals of cancer to urban planners being able to steer transportation systems in real time, the dream of instantaneous machines is embedded in the exemplary use cases. In this paper we argue that this prevailing imaginary misses an important ethnographic affordance of machine learning techniques. Namely, their ability to create friction. Taking inspiration from ethnographic fieldwork and pragmatist philosophy, we define moments of friction as encounters between differences that hold the potential to creatively re-evaluate held beliefs and rearrange engrained conceptual schemes. Friction thus enables the form of ‘sense of dissonance’ that Stark (2011) has argued to be central to organizational innovation and creativity. When we argue that computational methods can spur this form of dissonance, we actively seek to decouple data and algorithmic techniques from connotations such as ‘speed’ and ‘control’ and illustrate their potential as tools that can also further an ethnographic interest in furthering slower and more reflexive practices in organizations. Our paper thus contributes to the recent debate concerning the ways in which AI should (or should not) be integrated in the toolkit of professional anthropologists. Recently Artz (2023) provided a speculative list of 10 ways that AI will disrupt anthropology. Although we are aligned with many of his arguments – such as the need to think of AI as collaborative partner – our focus in this paper is more on the possibility to use computational methods to generate productive friction than their ability to reveal hidden cultural patterns.
Drawing on our 10 years of experience working with participatory data design (Jensen et al., 2021) and data sprints (Munk et al., 2019), we present a typology of data-frictions that each contribute to ‘slowing down reasoning’ (Stengers, 2018) in distinct ways. We first outline a conceptual framework around data frictions. We ground the concept in Agar’s argument that the decision to engage in ethnographic fieldwork always involves putting your own point of view at risk (Agar, 2006). In fact, the insight that your own cultural frame of reference may not be sufficient to describe the world is what motivates fieldwork in the first place. We also take inspiration from the pragmatist theory of inquiry stemming from Charles Peirce (1878) and John Dewey (1929). Subscribing to a processual ontology both entertained the idea that relevant knowledge about the empirical world is produced in ‘problematic situations’ that in one way or another trouble existing classifications and habits of thinking. In other words, situations that create friction. We use the name ‘machine anthropology’ (Pedersen, 2023) to denote a specific type of humanistic data science that actively seeks to repurpose algorithmic techniques to produce such frictions.
We then exemplify the practice of machine anthropology across three cases where we have worked with applied anthropologists who found themselves enmeshed in two types of frictions while working with algorithms. One type is ‘emic frictions’ that challenge their empirical hypotheses. The other is ‘etic frictions’ that provoke wholly new ways of theoretically framing the problem at hand. In The Thick Machine (TTM) we invited ethnographers to compete with a neural network in interpreting the meaning of emoji reactions on Facebook. In the case of PUBLIKUM, we collaborated with Will&Agency (W&A), a consultancy, in using unsupervised algorithms to explore whether the movie scripts they develop with customers in the industry resonated with online discussions. In ‘Do you live in a Bubble?’ (DYLIAB) we worked with Gehl Architects to create cartographies of Copenhagen’s political diversity through patterns in digital traces from Facebook users. As we will show below, these cases illustrate how both emic and etic machine friction have the potential to stimulate creative thinking and disrupt established beliefs, fostering new insights and perspectives.
We thus introduce the idea that algorithmic techniques from data science can be re-appropriated as ‘friction machines’ if they are liberated from specific quality-criteria associated with the framework of acceleration and control they are usually embedded in. With roots in the cases, we distinguish between three types of friction machines that each trouble a specific criteria in said frameworks. ‘Algorithmic friction’ thrives on mispredictions and thus troubles the idea that machine learning techniques should be evaluated on their accuracy. ‘Visual friction’ thrives on forcing the user to engage with data visualization in order to make sense of it. It thus troubles the idea that data visualizations should ideally be easy to interpret and tell a clear and unambiguous story. ‘Curated friction’ thrives on the indeterminacy in operationalization and thus troubles the idea that the goal of data science is to ‘mine’ and represent pre-existing structures in data. Rather data-work is a processual task of deciding how to perform such structures with the techniques at hand. We end the paper by linking the three types of friction machines back to David Stark’s theory on the relations between dissonance and innovation.
What is data friction?
There can be no ethnography without friction. It is fundamental to ethnographic methods that a translation takes place between two points of view and that this translation is fraught with the kind of pitfalls that result from ethnocentrically taking a specific framing of the world for granted (Agar, 2006). Friction, then, is what you get when you actively seek to unsettle those taken-for-granted assumptions through fieldwork. Hammersley and Atkinson (2019) talk about fieldwork as apprenticeship and seeking out a position as the acceptable novice. The moment you get too comfortable in your role – the moment it gets frictionless – fieldwork is no longer productive and it is time to seek out a new position. A similar line of thought can be traced back to pragmatist philosophy and the argument that productive inquiry stems from so-called ‘problematic situations’ where existing classifications are misfits with empirical reality (Dewey, 1938). As the world changes, our conceptual schemes need adjustments. However, this is only possible with a certain amount of friction between existing schemes and the empirical world. As put by Peirce, you need an epistemic ‘itch’ that motivates further innovative inquiry and thus keeps you on your toes when it comes to adjusting your frame to an ever changing environment (Peirce, 1877). While initially annoying, such itches are necessary in the production of knowledge. According to Stark (2011) they are also necessary for maintaining innovative and competitive organizations.
Taking further inspiration from ethnography we distinguish between what we will here refer to as emic and etic frictions. Emic friction is what occurs when you realize that you are a stranger to your field. It begins with the initial realization that your interlocutors live in a different frame and thus experience the world from a different point of view that makes translation necessary. The friction is between your own point of view and theirs. This is the itch that typically motivates the ethnography: it becomes clear that your way of seeing the world is not compatible with your interlocutors’. Fieldwork, then, is to a certain degree about seeking out opportunities to further increase this emic friction in order to learn from it. Etic friction, on the other hand, is when you realize that it is not enough to learn about your field but that you will have attune your own conceptual frame to grasp what you are dealing with. Any good ethnography, one could argue, involves some level of iterative attunement between emic and etic frictions. This idea is represented in the first columns in Figure 1 below.
Seen in this light, it is perhaps not surprising that the conventional narrative about data science as an accelerator for insights and vehicle of control can appear fundamentally incompatible with ethnography. Often marketed as tools to circumvent cumbersome theory and reduce friction, one can hardly blame anthropologists for harbouring a certain scepticism towards such techniques. One example is the imaginaries around the use of AI in creating the so-called smart city. Here, the selling point has been to model and track urban flows in real time and thereby reduce traffic jams and other infrastructural problems (Kitchin, 2014b). You could say that AI has been used to avoid the loss of efficiency in mechanical motion of objects moving through the city (Madsen et al, 2022). Similarly, machine learning in the film industry is often envisioned as a powerful tool to optimize box office success (Sharda & Delen, 2006), i.e., a way to ensure that time and resources are not wasted on movies that lack commercial potential. This could be dubbed the Hollywood-model of creative AI, but it is not necessarily creatively acceptable AI. Finally, we have seen large social media platforms taking advantage of people’s digital traces to enable targeted news circulation in real time. We could call this the Cambridge Analytica approach to digital traces, but it is far from the only use one could have of such data.
The red thread across these three examples is that data and algorithms are used for optimizing processes towards reaching pre-defined goals. They speed up answers to established questions and the frames behind those questions are not up for scrutiny. For instance, the smart city’s ambition to model the efficient flow of cars in the city is ultimately rooted in a conceptual model positioning the existence of a continuous geographical space (Madsen, 2023). Without this frame it would not make sense to model traffic in the ways it’s done in smart city projects. However, in the smart city there is often no ambition to disturb that spatial frame. Rather, the aim is to find an efficient answer to the question concerning how to make cars move in said space (Halegoua, 2020). What is at stake is the empirical hypotheses derived from the spatial frame – not the frame itself. The search for solutions stays within the original ‘point of view’. In the traditional framing of data science, data-friction is something to be avoided and we illustrate this approach in the middle column of figure 1 below. To continue the smart city illustration the epistemic itch here may be felt by a municipal traffic engineer whose point of view is that the good city is one that efficiently moves cars from A to B (PoV1) but fails to predict these car flows (epistemic itch). His solution is to invest in a combination of sensors and machine learning models that enables him to better predict the car pool (algo as revealing a pattern). This removes the itch and the engineer returns safely to PoV1 without even having to encounter other point of view on the good city (poV2).
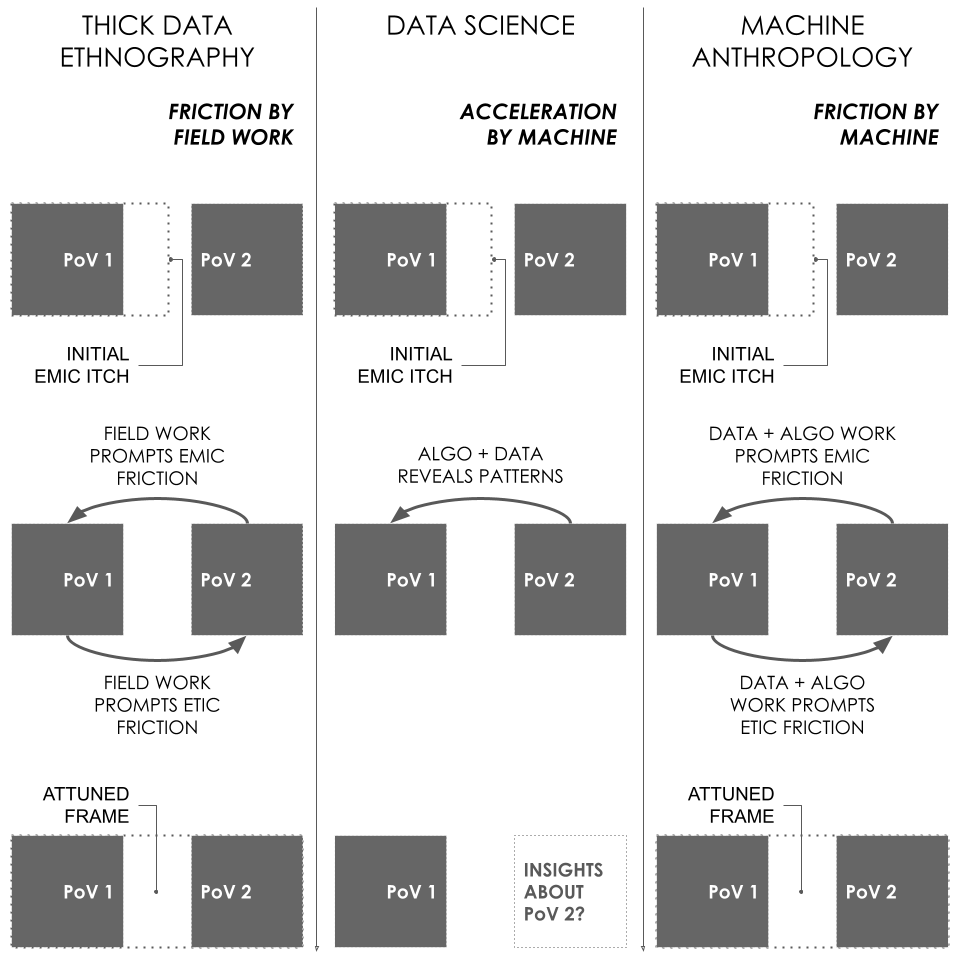
To the contrary, we propose that there is a way of working with data and algorithms that produce both emic and etic frictions (Figure 1, Right). A form of data science that does not see data frictions as problematic but rather as a potential instrumental for doing something ethnographic with data science methods. It is an approach to data science that actively seeks to foster moments where algorithmic pattern-recognition makes different problem frames rub against each other and thus stimulate the same form of attunement as classic fieldwork does. Our suggestion is that we can be more innovative in our uses of data science techniques if we learn to re-appropriate algorithms as ‘friction machines’. As techniques that help us rethink our problem solving strategies at the right moments in a process of inquiry. It is important to emphasize that we do not think of such productive data-frictions as something that arises spontaneously. Most people prefer to avoid friction and safely rest in their native point of view, so frictions have to be deliberately designed and cared for. Just as traditional anthropology has invented methodological techniques to avoid the risk of ‘going native’ (Fuller, 1999), so will machine anthropology have to develop a typology of productive data-frictions and device strategies for realizing them.
THREE EXPERIMENTS IN MACHINE ANTHROPOLOGY
This section introduces three case-studies where we have experimented together with ethnographers and consultants in practicing machine anthropology within their fields. In The Thick Machine (TTM) experiment we built an arcade game where ethnographers could compete against a neural network in guessing emoji reactions on Facebook (Munk et al., 2022). In the case of PUBLIKUM, we collaborated with Will&Agency (W&A), a consultancy firm, to develop a platform that would enable producers in the movie and television industry to examine how their movie themes resonate with ongoing societal discussions. In ‘Do you live in a Bubble?’ (DYLIAB) we worked with Gehl Architects to create cartographies of Copenhagen’s political diversity through patterns in digital traces from 150.000 Facebook users (Madsen, 2022). Together these cases will illustrate how emic and etic machine friction have the potential to stimulate creative thinking and disrupt established beliefs, fostering new insights and perspectives. For each, we will a) describe the empirical ‘itch’ that motivated the experiment, b) elaborate on the prior held beliefs existing amongst the involved participants before the experiment, c) provide a detailed account of the data techniques used and d) discuss the emic and etic frictions it gave rise to.
The Thick Machine
The motivation for designing a game where ethnographers could compete against a neural network in guessing emoji reactions on Facebook was to test if machine learning would be able to interpret cultural meaning in online debates. Having experience in analyzing such debates qualitatively, TTM was essentially an experiment with our own practices as digital anthropologists. This involved risking prior beliefs about the respective strengths and weaknesses of thick data versus big data. For example, we presumed that there would be situations where a machine learning algorithm would be able to do as well as, or even better than, a human ethnographer in learning to behave like users of an online platform, but that these situations would be limited to things like emoji reactions where the infrastructure of the platform (in this case Facebook) provided a consistently datafied target and feature set for training. What motivated us to build the Thick Machine was thus an opportunity to test the extent to which state-of-the-art predictive machine learning (anno 2019) would be able to do interpretive ethnographic work.
We chose to work with neural networks because this ML technique was known to have the highest predictive accuracy at the time of the experiment. The neural network was trained on a large corpus of Danish language debate (posts and comments) on public Facebook pages. When a user commented and emoji reacted (‘heart’, ‘love’, ‘haha’, ‘wow’, or ‘angry’) on a post, the neural network was fed the emoji reaction as target and the tokenized comment text as features. It thus learned to associate particular patterns in comment text with a particular emoji reaction. The training took place on a randomly selected 70% of the corpus, reserving the remaining 30% for testing. In the arcade game, which we built as a physical device out of plywood, a screen, and a pair of Rasberry PIs, ethnographers in our lab were then invited to try their luck against the neural network guessing the correct emoji reactions from the post-comment pairs in the test set.
When the arcade game had been physically displayed on the lunch table in our lab for a week, encouraging our colleagues to play, we began evaluating the results. The most obvious thing to compare, we thought, would be the overall accuracy of the human players versus the machine. Somewhat surprisingly, the machine and the human players were equally bad. The machine had an overall accuracy of 51% (random would have been 20% with five equally distributed emojis to choose from), which we perceived as decent or at least a success to the extent that it clearly managed to get something right. This meant that there was, at least in some situations, discernible textual patterns associated with a type of emoji reaction that an algorithm could learn. What was more surprising to us was that our colleagues who had been playing the game against the machine had an almost identical overall accuracy of 52%. We therefore decided to explore the results as a confusion matrix to see which emoji reactions the human players and the machine respectively had a tendency to get right and wrong. This visualization became the central data artifact in the experiment.
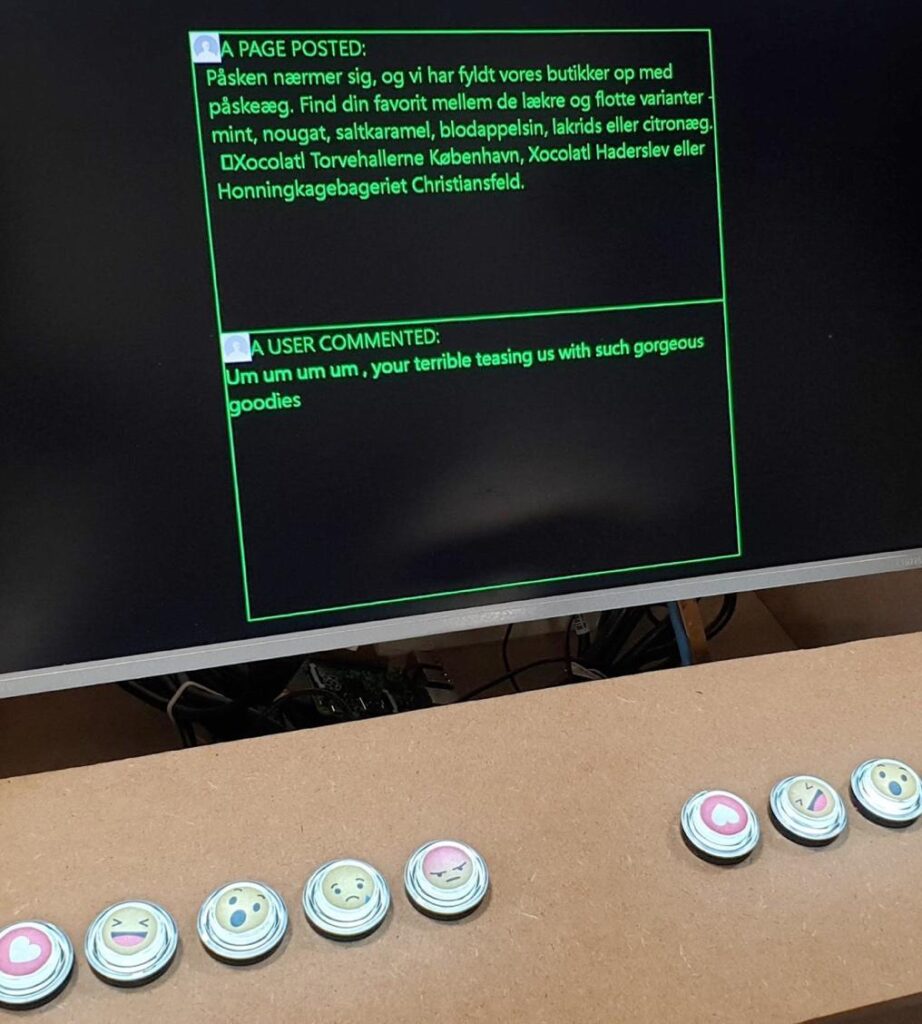
What kinds of friction are generated?
The gamification of the predictions in a sense ensured immediate buy-in from the participants in terms of making emic frictions visible. As a player of the arcade game you first see a randomly selected post from the test data followed by randomly selected comments to that post. You then commit to a prediction by pressing a button corresponding to an emoji reaction and the screen records your choice. When you are subsequently shown first the prediction of the neural network, i.e., your competitor, and then the correct answer, i.e., how the user who wrote the comment on Facebook actually emoji reacted, you are naturally prompted to reflect on why you guessed wrong, why you were right and the machine was wrong, or vice versa. Exploring the results of all the games as a confusion matrix allows you to put those reflections into perspective, for example by noticing that love reactions tend to be easily predictable by both the human players and the machine, or that wow reactions are really ambiguous and often mistaken for various other emoji reactions. Again, typically in the same way for both humans’ players and machine. You could say, then, that the algorithmic experiment facilitates emic friction with emoji culture on Facebook. Through the game you kind of empathize with the struggles of the algorithm to get certain situations right because you experience those difficulties alongside it.
The experiment forced us to realize that we were, in fact, unreflectively reproducing an ethnoscienetific idea about cultural algorithms. However, another layer of algorithmic friction became evident when we explored the confusion matrix and reflected on the nature of the game we had created. Was the point, as we had first imagined, to test if a neural network could “hang out” (i.e., train) in a Facebook data world and thus learn the cultural codes that govern how you emoji react in different situations? If so, then there were two ways of evaluating the game. Either the human players and the machine were competing against each other to become as good as possible at mimicking users on Facebook, or the machine was competing against itself to become equally bad (or good) as the human players (supposedly the real digital ethnographers) at mimicking the users on Facebook. In the former case, neither the machine nor the humans are doing particularly well. In the latter case, the machine is actually remarkably good at making the same mistakes and getting the same emojis right as its human counterparts. But the confusion matrix also manifested some etic friction here. If, in fact, a neural network was able to reproduce the same mistakes as digital ethnographers encountering a Facebook discussion for the first time trying to interpret what is going on, then perhaps the game is not actually a competition to be as accurate as anyone. Rather than thinking about the neural network as a device that would be able to perform cultural interpretation, i.e., do thick description, we began thinking about it as a device that would point us to situations worthy of said thick description.
There was, we realized, something essentially ethnoscientific about the way we had first devised the game. It was built on the presumption that there would indeed be discoverable cultural codes – or cultural algorithms – that governed how emoji reaction was done on Facebook. But the point of thick description, in Geertz’ original definition, was precisely to oppose that idea and show how some situations are deep with multiple, partially overlapping layers of meaning, complicating interpretation, and making thick description both necessary and worthwhile. It was, Geertz contended, precisely in the situations where there was no single ground, no basic cultural facts to explain unambiguously what was going on, but rather multiple emic readings of the same situation that interpretive ethnography was most needed. When you explore our confusion matrix and the underlying qualitative data it becomes evident that both digital ethnographers and the neural network encounter those deep situations in the same way, namely as a difficulty to predict the correct emoji reaction. The experiment thus generates both an emic friction with the users on Facebook, which points to potentially fruitful and worthwhile situations for thick description, and an etic friction with our own tacit understandings underpinning the design of the game.
Publikum
In the case of PUBLIKUM, our collaborators’ emic itch was to utilize “big social data” in an anthropological fashion, wherein the data could be employed to challenge both their own and their client’s (filmmakers) ethnocentrism regarding a script’s thematic alignment with ongoing societal discussions. This responds to a heightened sense of enthusiasm within the cultural industry to mobilize burgeoning reservoirs of social and cultural data.
Our collaborators initially harboured the belief that the PUBLIKUM (‘audience’ in English) platform could serve as a valuable tool to expedite their project tasks by swiftly identifying patterns within vast quantities of social media discussions. Initially. On the other hand, their clients, film makers and cultural actors, were driven by a sense of curiosity and anticipation. They saw the platform as an opportunity to actively engage with how a broader audience articulates and describes the thematic elements they are currently working on. Engaging with the platform was perceived to enrich their understanding and provide valuable reflections that could inform their manuscript writing process moving forward.
The PUBLIKUM platform facilitates the creation of semantic networks through a single click of a button, achieved by uploading data into an algorithm. This data is sourced from an external provider, wherein consultancy workers develop specific Boolean search strings tailored to each specific movie. As a result, movie-specific networks revolving around particular themes are generated. Leveraging natural language processing (NLP), the platform employs techniques such as Part of Speech (PoS) tagging and TF-IDF ranking to extract relevant words and establish connections when they co-occur within the same documents. To visually represent these interconnected keywords, visual network analysis techniques such as Force vector layout and Louvain modularity are employed (Jacomy et al., 2014). This approach enables the clustering of related keywords and distinguishes them visually using colour coding. The platform generates networks that provide visual depictions of ongoing onlife discussions (Floridi, 2015). These networks can be explored via an interactive dashboard, allowing users to click on individual keywords and access the sources that mention them. Consultants can thus delve into the exploration process and make annotations concerning the diverse ways in which a particular topic is discussed.
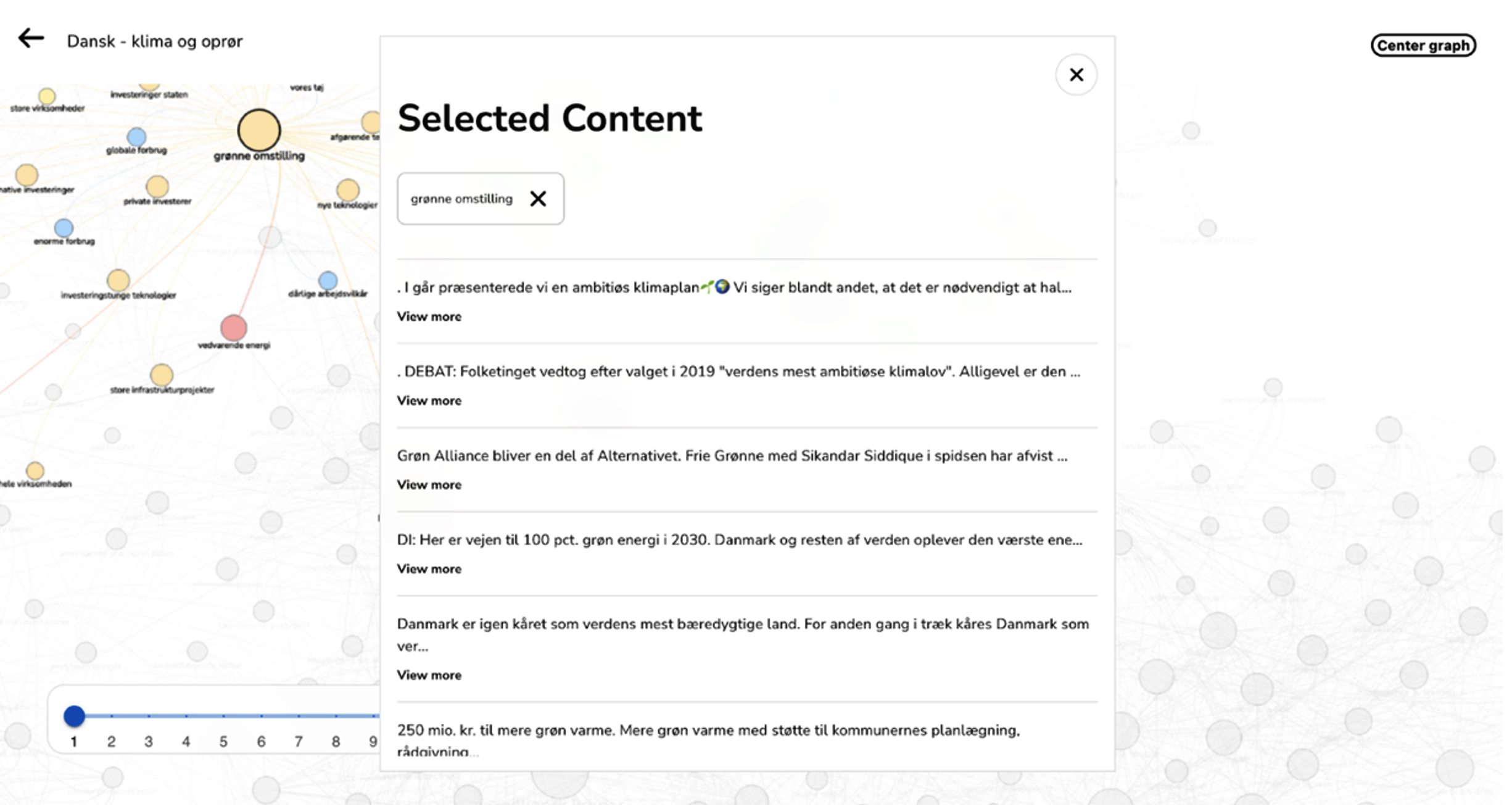
What kinds of friction are generated?
The PUBLIKUM platform, despite its one-click network generation function, did not expedite project tasks. Rather, consultants had to engage in intricate work of deciphering between worthwhile and subpar data, and between what would be generic findings or those valuable for further analysis. Initially, the act of downloading data from a data provider appeared straightforward, as Boolean search strings can be utilized to formulate search queries that assist in obtaining relevant information. However, in practice, this process required considerable attention, experimentation, and continuous refinement. Consultants encountered the challenge of translating a thematic concept, such as “youth climate rebellion,” into a search string that accurately generates the desired thematic outcome. To accomplish this, they must meticulously choose which words to incorporate into the search string, considering the point at which a word becomes too broad and yields irrelevant data. This endeavour may elicit frustration, as an incessant cycle of novel keyword attempts, inclusion, exclusions, and the pursuit of more refined equivalents in lexical compendia ensues, all aimed at identifying the words that most aptly encapsulate the discourse. However, through this frustration the consultants also began to care and learn about their data, e.g., understanding the language used in a specific context. In an anthropological fashion, they acquired the language of the community that they studied. Additionally, engaging with the PUBLIKUM platform necessitates a rigorous qualitative and quantitative process, involving continuous alternation between distant reading of networks and close reading of blogs, newspaper articles, or social media comments (Jänicke et al., 2015). This approach consumes a significant amount of time, but it is indispensable for extracting meaningful insights related to specific movies.
The observation of the consultant’s work illustrates that rather than a pure device of acceleration, PUBLIKUM engendered anthropological encounters with the online communities under investigation. Consultants had to combine their existing understanding of themes in order to carefully attune the search strings to capture all relevant, but not too generic data. Tinkering with search strings must therefore not be seen as a pure auxiliary task of arriving upon a pre-confined set of data, but as a creative process of demarcating what might be meaningful for the given movie theme. Friction in this case does not emerge in situations where data analysis is concluded but permeates the data gathering and analysis. Consultants are required to engage with search strings and therefore produce meaningful insights. The machine’s idiosyncrasy of potentially collecting too much/too little or ir/relevant data acts as a friction in their creative process. The ‘right’ search string is thus an outcome of calibrated friction.
Furthermore, observing the work of consultants, we found another type of machine friction. Insights and annotations derived from exploring the platform had the potential to introduce emic friction among cultural actors. This happened when creative writers or directors observed the various ways in which the topic, they were working on is discussed. It is not only one way in which their theme is described in the data, but multiple. A friction emerges where patterns within the text fail to disclose a singular and stable definition of a theme. Instead, they present multiple, heterogeneous, and unstable interpretations. This friction challenges the preconceptions held by creative directors and manuscript writers regarding the themes, as well as their expectations of how the potential audience will respond to the film.
The visualization of networks and the multiplication of themes also leads to the emergence of etic frictions. This can be exemplified by a workshop among consultants, directors, and producers that we participated in, involving the development of a network encompassing a corpus of 174,323 online discussions focused on social housing areas in Spain. One annotation drew attention to the vibrant nature of these areas, characterizing them by the presence of youthful creativity, artistry, and a flourishing music scene. The producer involved in the workshop expressed her concern regarding this annotation, because she had repeatedly insisted the protagonist should be a middle-aged woman that she considers notoriously underrepresented in large-scale television productions. The tension between the annotation and her vision for the movie led to a moment of silence during the workshop, followed by a negotiation of what subsequent steps to take based on these findings. The consultants had successfully created a friction between the data and the producer’s ideals. After some deliberation on how to interpret the findings, the author of the manuscript offered an alternative reading, suggesting that the concept of ‘youth’ could be reframed according to experiences of being new to a country. As a consequence, the created friction as well as the moment of deliberation allowed for a deeper exploration of the experiences within this community, which consisted of diverse immigrants. Such an alternative perspective had the potential to offer a unique understanding of the norms and dynamics of the host country. What initially sparked an emic friction, evolved through the discussions among all participants involved in the workshop, leading to an etic friction. The focal point of this friction shifted as “youth” underwent ontological redefinition to become something that was both useful and productive for their story.
This episode illustrates how network visualizations acted both as a challenge for movie producers while also initiating moments of productive negotiation. Machine friction thus created a site of creative practices. While in this case causing a moment of disconcerting for the producer, visualizations that yielded no challenges to preconceived ideas were generally considered not as meaningful by the filmmakers. Providing frictionless or generic insights lead to frustration whereas some visual friction with the networks allowed for negotiation. The objective for the consultants lies therefore in generating the right friction and subsequently orchestrating workshops that open spaces of re-configuring themes and topics.
Do You Live in a Bubble?
DYLIAB was a project initiated because urban planners have a hard time mapping the ephemeral issue of political diversity in urban life. The only available data on urban political geography is the voting statistics that are aggregated on electoral districts by the national bank of statistics. Besides that, the main source of knowledge is hearsay in the sense that people living in cities have their own mental maps of where they fit in politically or not. This was the ‘itch’ that stimulated the need for experiments. The architects turned to data from Facebook to map the city because their personal mental maps did not carry enough weight to act as consultants on the issue and the available data was too narrow in space and time. None of the available tools seemed satisfactory in terms of understanding how urban life influences political segregation on the scale of the city. The hope was that data from Facebook’s API would provide a quick answer to a question that would otherwise be difficult to approach. A quick gateway to understand the city from the perspective of the 150.000 users that interact with political and urban events in Copenhagen on Facebook.
The architects know Copenhagen both as residents and professionally as urban planners. They thus expect some areas and streets to be low on political diversity and others to be high. Knowing Copenhagen, many in the project expected the area of Nørrebro to be low on political diversity and each of the participants held beliefs about the extent to which their own streets and areas would have a high or low political diversity. These beliefs were all grounded in a shared assumption that urban spaces can be translated into a continuous area on a geographically projected map. Under this assumption an area could be a given street, a public square or perhaps a neighbourhood. Each area could then be assigned a political diversity score.
The artifact we built for acquiring this new point of view was an explorative database where each venue in Copenhagen had a diversity score represented as a dot on the map, which was constructed in the following way. First, we assigned a political orientation to anonymous users’ ID based on their interaction with political content. For instance, if a user was disproportionately positive towards content from left wing content, he/she would be marked as ‘left leaning’. Second, we looked at the attendance-lists of 120.000 events in Copenhagen and cross-referenced it with the first list of IDs to describe the political breakdown of the event. For instance, if a concert had 100 people attending, we would describe the percentage of these belonging to the left wing, the economic liberals, and the nationalist parties respectively. Third, we translated this distribution into a diversity index between 0 and 100, where 0 indicates an event that could only attract people from one political block whereas 100 would indicate a diverse event where the audience was split between the three blocks. It was from this basis that we produced the local cartography of political diversity that can be found here: www.tantlab.aau.dk.
By clicking on the dot, you can make the political breakdown of the venue as well as a description of its most politically diverse event. Importantly, the granularity of the underlying data means that the datascape could have been built in various ways. An important part of constructing it was thus to align on decisions as to how to curate the data and to what extent we would use algorithmic techniques – such as spatial autocorrelation – to find patterns in it. We ended up building an explorative datascape on a geographical map that enabled the architects to ‘hunt’ for patterns rather than receiving those patterns from an algorithmic segmentation.
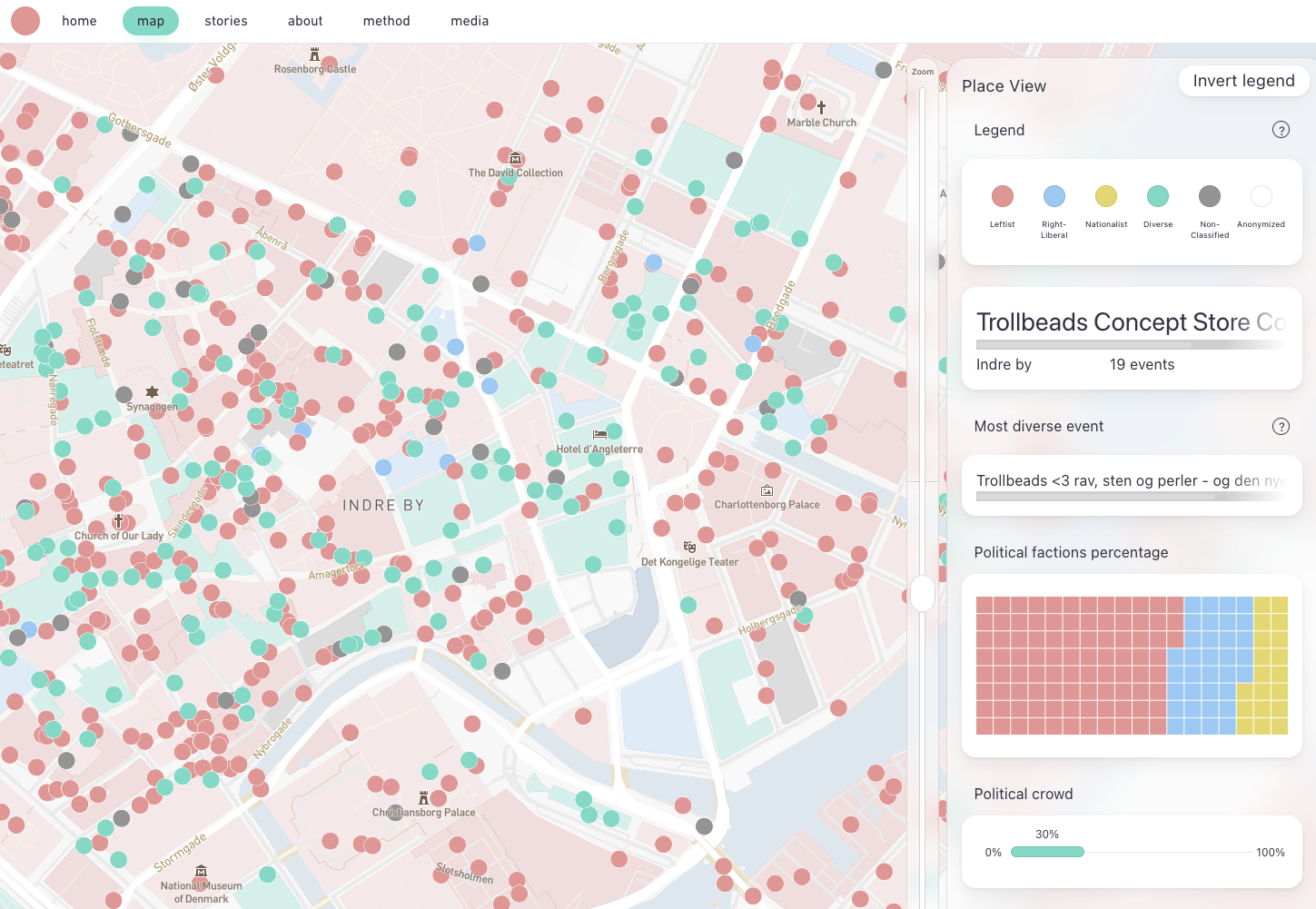
What kinds of friction are generated?
As an artifact this datascape afforded emic frictions by returning different results than expected by the architects. In their first data explorations, conversations sounded as follows. Why is Israel’s Plads low on political diversity? It’s been designed for such a variety of uses? What about the waterfronts? Why is Amager beach high on diversity whereas the harbour front is low? Does that have something to do with the accessibility of public transport? Is political diversity explained by transport patterns rather than architecture? All these questions are examples of emic frictions where the point of view of the architects are in dissonance with what they see on the map. However, since much of the map makes sense, they feel committed to explore these frictions and provide explanations for them. However, the datascape also stimulated etic frictions around theoretical commitments concerning the role of space in public life. Whereas the questions above make sense within the frame of continuous space discussed above, some patterns in the datascape troubled this. An example is the tendency for types of venues such as tattoo parlours, football stadiums and specific food venues to stand out as especially diverse. Perhaps they even share many of the same. Being spread across town; they do not constitute an ‘area’. They are distant in geographical space – but may be socially proximate to each other socially. This troubles Tobler’s law stating that “everything is related to everything else, but near things are more related to each other”. While this is a rule that does have a big impact in architecture and urban planning the data stemming from Facebook affords representing space in different ways than geographical. For instance, the architects embarked on visualizing this data as a network where two venues were close if theory shared many users. An experiment that moved them from emic friction to etic frictions that troubled important beliefs in social geography.
BUILDING FRICTION MACHINES
The three presented cases above all share the ambition to use AI and Big Data as devices of friction rather than acceleration. We refer to this as the art of building friction machines. However, it is important to emphasize that such machines can be built in a variety of different ways depending on the context of use. As illustrated in the table below each case has its friction machine built in a distinct way as a response to a distinct epistemic itch. For instance, whereas both TTM and PUBLIKUM involve the use of machine learning, DYLIAB puts more emphasis on visual exploration.
We propose to think of the different data techniques used as different building blocks that can be combined in distinct ways to produce predictive frictions. In fact, we want to argue that the competence to build friction machines that fit a given organizational context and ‘itch’ is central to the profession of the machine anthropologist. In the last part of this paper, we therefore want to suggest a mini typology of friction machines that can perhaps inspire future experiments in this area. Each of these types have reference back to the three cases above and each of them entails rethinking and sometimes abandoning central concepts and suggestions for best practices inherited from the ‘acceleration framework’. We think of these types as data-intensive equivalents to the methodological tactics anthropologists have developed to maintain productive friction while doing fieldwork, such as seeking out situations of acceptable apprenticeship, making sure to actively shift situation when things become taken-for-granted routine, or using introspective fieldnotes to reflect on your own position in the field. They are useful for the anthropologists precisely because there is a constant risk of ‘going native’, i.e., a risk of avoiding friction with a field that thus becomes a docile setting. The equivalent risk in machine anthropology is to slide back in the quality-criteria associated with the accelerated data science framework in figure 2. The three types of friction machines discussed below are proposed as remedies to that risk.
Table 1. Comparison of Case Studies: DYLIAB, Thick Machine, and Publikum
Case | Prior Held Beliefs | The Initial ‘Itch’ | Data Technique | Emic Friction | Etic Friction |
DYLIAB | Anecdotal evidence on the political diversity of specific Copenhagen areas | The hope that Facebook traces can offer relevant data on a more granular scale than voting patterns in electoral districts | The production of a diversity index and explorative datascapes designed from | Surprises regarding the political diversity of specific areas and a suggestion to move the focus from urban design to urban mobility in explaining political diversity | A need to revisit theories of space underlying the original beliefs. A move from Tobler’s law and geographical proximities to networks and social proximities. |
Thick Machine | Assumptions about machine learning ethnography and thick data vs big data. | The hope is to test how good a neural network performs compared to a human ethnographer online. | Confusion matrix | Surprise about which emoji reactions are easy and difficult to interpret on Facebook. | Rethinking what machine learning can do for thick description. From doing the interpretation (high accuracy) to pointing us to worthy situations that are deep enough (through its failures) |
Publikum | Initial research and personal experiences related to writing a movie script. | The hope is to reach a larger audience and/or secure more funding for their creative project. | The production of a visual network that explores different ways of thinking about movie thematics. | Multiplication rises, when a topic is revealed not to have a single stable definition, but multiple heterogeneous, and unstable interpretations. | Rethinking core thematics aspect of the manuscript. A move from personal ideas to ontological reflections and negotiations. |
Algorithmic Friction (and the Reformulation of ‘Accuracy’)
One strategy for creating data-frictions is to commit to letting machine learning algorithms find patterns in complex datasets. As formalized program machine learning algorithms have the specific affordances of a) putting things into relation with each other in a structured way and b) returning such relations in charts and numbers. An example of how this can stimulate productive frictions is the use of predictive neural networks in building the think machine. The algorithm has a structured and formalized way of relating training data encoded by humans and algorithmic predictions on the same data. Moreover, the confusion matrix returns these relations in a way that can be accessed and evaluated by the participants. The relation manifests itself as false positives and false negatives and a measurement of the accuracy of the applied algorithmic more. In TTM the confusion matrix served the role as an artifact that prompted participants to rethink what machine learning could/should do in interpretative ethnographic work. Instead of finding neural networks relevant because of their high accuracy, participants were stimulated to think about the failures of the machine, its thickness (in the sense of being daft), as an asset that could point to deep situations worthy of actual thick description (in the sense of constructing a reading of deep situations where multiple layers of meaning make interpretation complicated and a moving target).
However, while the confusion matrix – used in this way – has the potential to be a component in a friction machine it is not usually presented as such. In order to serve this function its core components need reinterpretation. It needs to be liberated from its usual use in the accelerated frame. In the thick machine this is done by turning the notion of ‘accuracy’ on its head. Instead of interpreting low accuracy as a reason to discard the algorithm, algorithmic misprediction is used as a tactic to identify ambiguous and rich cases where thick description is necessary and worthwhile (Munk, Olesen & Jacomy, 2022; Rettberg, 2022). Something similar is happening in PUBLIKUM where the use of TF-IDF techniques liberated from the attempt to identify the ‘correct’ amount of topics in the qualitative material. Rather, the verdict on the relevance of the returned topics is something that is established in dialogue with the formalized algorithm which is naturally biased toward prioritizing differences across documents. The relevant topics are the ones that point to discussions that are deep enough to serve as inspiration for creative manuscript writing. This use of techniques of algorithmic pattern recognition requires a new way of talking about – and evaluating – their epistemic qualities. Instead of thinking about such techniques as marking ‘the end of theory’ (Anderson, 2008) and the rise of pure inductive science, we propose to think of them through the lens of ethnography and pragmatism. We have already mentioned how they in a Geertzian frame can be thought of as guiding attention to situations in need of thick description. As an addition to that we want to propose that we can think of their guidance as an example of the mode of thinking that Peirce called ‘diagrammatic reasoning’ (Tylén et al., 2014). This strategy of inquiry is precisely characterized by letting collective attention guide by formalized systems in order to arrive at intersubjective moments where frictions between points of view are explicated. In the context of machine anthropology, we suggest thinking about machine learning from this philosophical backdrop rather than any proposition as to how such techniques can lead to empirical investigations free of theory and bias.
Visual Friction (and the Reformulation of ‘Design Guides’)
The second friction strategy relies less on algorithmic data-processing and more on designing artifacts to support visual thinking. Whereas algorithmic friction returns clear numbers that can very clearly disturb pre-existing expectations if one knows how to interpret them, visual friction requires less data literacy. Feeding on developments in interactive data visualization it involves building datascapes that enables users to collectively explore granular and multifaceted datasets in ways they deem interesting. Both PUBLIKUM and DYLIAB takes advantages of this possibility by involving the applied anthropologists in conducting visual filtering on a broad set of metadata and zooming between aggregated overviews and qualitative details. The datascapes thus come to function as a semi-structured sandbox where tactics for navigating and interpreting complex data become a collective concern. The effect is that people’s distinct navigation tactics are put in relation to each other in ways that generate productive frictions.
However, using data visualization as a component in a friction machine also requires revisiting and rethinking some of the dominant guidelines for how to produce them. This is especially true for guidelines associated with the so-called explanatory data visualization that we know from e.g., data journalism. Here the aim is to produce visualizations that efficiently communicate an already established truth (Dzuranin, 2022). This involves a simple and structured narration of which the genre of scrolly telling is a great example. To the contrary the explorative datascapes in both PUBLIKUM and DYLIAB are built to indicate vague structures (patterns of dots or clusters) that require more investigation to be meaningful. In that sense they stimulate explicit guessing and sense-making in the presence of others. An activity that can even be further stimulating by deliberately hiding labels and other information before guesses are made.
This is a way of thinking about data visualizations can once again find its theoretical home in ethnography and pragmatism that both emphasize the need for developing techniques to stimulate a form of abductive reasoning where the practice of guessing plays a central role (Tschaepe, 2014). Importantly, abductive thinking does not thrive on guessing run wild. In his writings on the topic Peirce emphasized the need to stimulate what he called controlled and piecemeal guessing. He insisted that there is a normativity to the practice of guessing in the development of hypotheses – the fundamental aim is to motivate people to make sense of specific tangible empirical patterns. Our suggestion is that we can productively think of interactive data visualizations as serving this aim rather than the aim of telling clear and unambiguous stories. This can, for instance, be achieved by deliberately hiding information to make the visual artifacts vague enough to stimulate guesses (Munk, Madsen & Jacomy, 2019).
Curated Friction (and the Reformulation of ‘Noise’)
The third friction strategy revolves around the curation of data and the operationalization of metrics. Because the contemporary data environment is way more granular and messier than its predecessors, data projects often involve decisions about what to keep as data points and how to operationalize concepts. This task is also a task of putting distinct data points into relation with each other by grouping some as relevant and perhaps even adding some together to produce a relevant measure. In the case of PUBLIKUM we saw how the task of demarcating relevant information from irrelevant data generated discussions about the scope of the inquiry and what would be, to the consultants’ minds, creatively acceptable to the client. Similarly, in DYLIAB it was the difficulty of aggregating the relevant dots in a continuous space that motivates the attempt to measure social distances and thereby trouble the notion of space altogether. The interesting frictions stem from the process of curating data and measurements.
However, both cases also illustrate that there is a need to rethink the notion of ‘noise’ in order to achieve this kind of friction. What counts as noise changes when etic frictions motivate a change in the original frame. In DYLIAB diverse venues were in the beginning seen a noisy in the sense that they disturbed the possibility to see where public space ensures diversity. However, once the spatial frame changed, the architects revaluated their notion of which data points counted as noise and which did not. A similar shift in the demarcation of noise from signal happens in PUBLIKUM as well. So, rather than thinking about data curation and cleaning as a matter of reducing noise it can be seen as a process of establishing demarcations around what is meaningful. Again, we can make sense of this through Peirce’s approach to ‘operationalist thinking’ which precisely suggests that one of the heuristic values of quantification is that it necessitates explicit conversations about this demarcation (Peirce, 1878, Dewey, 1929). Conversations that often bear with them etic frictions.
MACHINE ANTHROPOLOGY AS ORGANIZATIONAL INNOVATION?
After having outlined three types of data-frictions and rooted them in the academic fields of ethnography and pragmatism, one question remains? Why is it valuable for applied anthropologists to engage in building friction machines? Why turn to machine anthropology when traditional fieldwork can deliver both emic and etic frictions? In order to answer this, we return to Stark’s theory of innovation (Stark, 2011). In his book ‘the sense of dissonance’ he makes the argument that innovative organizations are characterized by incorporating heuristics for stimulating friction and dissonance in the right moments in a decision process. Importantly, his work emphasizes how formalized models can play such a role if they are repurposed in ways that liberates them from the logic of efficiency that often guides their production. More specifically, in his studies of financial trading he (and Beunza) introduces the notion of ‘reflexive modelling’ to describe situations where predictive models are repurposed by traders (Beunza, Stark, 2012). Whereas these models are originally designed to predict market dynamics, traders repurpose them to understand the social factors that shape the behaviour of other traders. Models are used as social cues rather than objective representations of the market. Traders recognize that their models are influenced by their own interpretations and assumptions, as well as the interpretations and assumptions of others. They come to act as devices for stimulating collective conversations about exactly that. They help avoid the taken-for-grantedness of traditional models.
Stark and Beunza’s point is that this ability to repurpose formalized techniques – and liberate them from their intended use – helps improve innovative problem solving among traders. Just as points can be generalized to other professions, we see our work as extending these ideas into data science in a broader sense. The suggestion of friction machines has similarities with Stark’s reflexive models, but our focus is more narrowly focused on how to design productive frictions through curation of granular data, experiments with pattern recognition and design of interactive visual interfaces. Just as reflexive modelling, machine friction necessitates asking searching questions and reflecting critically on the potential negative consequences of accelerated automation and uncritical AI adoption. By creating emic and etic friction through machine learning, underlying assumptions become visible and tangible, creating avenues for practitioners to rethink ideas and explore alternative approaches. Furthermore, machine friction advocates for adaptive practices. Instead of relying solely on established best practices and the acceleration framework, practitioners are urged to continually reassess and adapt in response to friction and dissonance created by the machine.
ABOUT THE AUTHORS
Anders Koed Madsen is associate professor and head of experimental practice at the Techno-Anthropology Lab. With a background in philosophy, Science & Technology Studies, and Internet studies, his research is built on three intertwined streams. Under the heading of ‘soft city sensing’ he is using social web data to maps and understand urban experiences. Drawing on pragmatist philosophy he rethinks the connotations around quantification within the humanities. Additionally, he explored the influence of unstructured data and algorithms on human perception and organizational decision-making.
Anders Kristian Munk is associate professor at the Techno-Anthropology Lab and scientific director of MASSHINE. He does research on controversies over new science and technology, for example in relation to artificial intelligence or green transitions. He has been particularly engaged in the development of new digital methods at the interface between data science and anthropology. He is currently interested in the establishment of a methods program for computational anthropology, particularly in light of recent developments in machine learning and generative AI, and in the methodology of digital controversy mapping.
Johan Irving Søltoft is an industrial PhD student at the Techno-Anthropology Lab and the Danish consultancy firm Will &Agency. He is involved in the integration of ethnographic and machine learning methods within the cultural industry. He has been particularly engaged in developing AI tools that creative professionals find both creatively acceptable and applicable.
REFERENCES CITED
Agar, Michael. 2006. “An Ethnography by Any Other Name…” Forum Qualitative Sozialforschung/Forum: Qualitative Social Research.
Anderson, Chris. 2008. “The End of Theory: The Data Deluge Makes the Scientific Method Obsolete.” Wired Magazine 16 (7): 16-07.
Artz, Matt. 2023. “Ten Predictions for AI and the Future of Anthropology.” Anthropology News website, May 8. Accessed August 31, 2023.
Beunza, Daniel, and David Stark. 2012. “From Dissonance to Resonance: Cognitive Interdependence in Quantitative Finance.” Economy and Society 41 (3): 383–417.
Bielo, James S. 2016. “Creationist History-Making: Producing a Heterodox Past.” In Lost City, Found Pyramid: Understanding Alternative Archaeologies and Pseudoscientific Practices, edited by J. J. Card and D. S. Anderson, 81-101. Tuscaloosa: University of Alabama Press.
Dewey, John. 1929. The Later Works of John Dewey, 1925 – 1953: 1929: The Quest for Certainty. Carbondale: Southern Illinois University Press.
Dewey, John. 1938. Logic: The Theory of Inquiry. USA: Henry Holt & Company, Inc.
Dzuranin, Ann C. 2022. “EXPLANATORY DATA VISUALIZATIONS.” Strategic Finance 103 (7): 42-49.
Floridi, Luciano. 2015. The Onlife Manifesto: Being Human in a Hyperconnected Era. Springer Nature.
Fuller, Duncan. 1999. “Part of the Action, or ‘Going Native’? Learning to Cope with the ‘Politics of Integration’.” Area 31 (3): 221-227.
Hammersley, Martyn, and Paul Atkinson. 2019. Ethnography: Principles in Practice. Routledge.
Harris, Marvin. 1976. “History and Significance of the Emic/Etic Distinction.” Annual Review of Anthropology 5 (1): 329-350.
Jacomy, Mathieu, Tommaso Venturini, Sebastien Heymann, and Mathieu Bastian. 2014. “ForceAtlas2, a Continuous Graph Layout Algorithm for Handy Network Visualization Designed for the Gephi Software.” PloS One 9 (6): e98679.
Jänicke, Stefan, Greta Franzini, Muhammad Faisal Cheema, and Gerik Scheuermann. 2015. “On Close and Distant Reading in Digital Humanities: A Survey and Future Challenges.” EuroVis (STARs) 2015: 83-103.
Jensen, Torben E., Andreas Birkbak, Anders K. Madsen, and Anders K. Munk. 2021. “Participatory Data Design: Acting in a Digital World.” In Making and Doing STS, edited by Teun Zuiderent-Jerak and Gary Downey, MIT Press.
Kitchin, Rob. 2014a. The Data Revolution: Big Data, Open Data, Data Infrastructures and Their Consequences. Sage.
Kitchin, Rob. 2014b. “The Real-Time City? Big Data and Smart Urbanism.” GeoJournal 79 (1): 1-14.
Madsen, Anders K. 2023. “Digital Methods as ‘Experimental a Priori’–How to Navigate Vague Empirical Situations as an Operationalist Pragmatist.” Convergence. doi: 10.1177/13548565221144260.
Madsen, Anders K. 2022. “Do You Live in a Bubble? Designing Critical Metrics to Intervene in the Cartography of Urban Diversity.” Projections 16.
Madsen, Anders K., Andreas Grundtvig, and Sofie Thorsen. 2022. “Soft City Sensing: A Turn to Computational Humanities in Data-Driven Urbanism.” Cities 126: 103671.
Munk, Anders K., Anders K. Madsen, and Mathieu Jacomy. 2019. “Thinking through the Databody.” In Designs for Experimentation and Inquiry: Approaching Learning and Knowing in Digital Transformation, edited by Åsa Mäkitalo, Todd Nicewonger, and Mark Elam, 110. Routledge
Munk, Anders K., Axel Meunier and Tommaso Venturini. 2019. “Data Sprints: A Collaborative Format in Digital Controversy Mapping.” In digital-STS: A Field Guide for Science & Technology Studies, 472.
Munk, Anders K., Asger Gehrt Olesen and Mathieu Jacomy. 2022″The Thick Machine: Anthropological AI Between Explanation and Explication.” Big Data & Society 9(1):20539517211069891.
Pedersen, Morten Alex. 2023. “Editorial Introduction: Towards a Machinic Anthropology.” Big Data & Society 10(1):20539517231153803.
Peirce, Charles Sanders. 1877. “The Fixation of Belief.” In The Pragmatism Reader: From Peirce Through the Present, edited by R.B. Talisse & S.F. Aikin, Princeton University Press, Princeton.
Peirce, Charles Sanders. 1878. “How to Make Our Ideas Clear.” In The Pragmatism Reader: From Peirce Through the Present, edited by R.B. Talisse & Scott F. Aikin, Princeton University Press, Princeton.
Rettberg, Jill Walker. 2022. “Algorithmic Failure as a Humanities Methodology: Machine Learning’s Mispredictions Identify Rich Cases for Qualitative Analysis.” Big Data & Society 9(2):20539517221131290.
Sapienza, Anna, and Sune Lehmann. 2021. “A View from Data Science.” Big Data & Society 8(2):20539517211040198.
Sharda, Ramesh, and Dursun Delen. 2006. “Predicting Box-office Success of Motion Pictures with Neural Networks.” Expert Systems with Applications 30(2):243–254.
Stark, David. 2011. The Sense of Dissonance. Princeton University Press.
Stengers, Isabelle. 2018. Another Science Is Possible: A Manifesto for Slow Science. John Wiley & Sons.
Tschaepe, Mark. 2014. “Guessing and Abduction.” Transactions of the Charles S.Peirce Society: A Quarterly Journal in American Philosophy 50(1):115–138.
Tylén, Kristian, et al. 2014. “Diagrammatic Reasoning: Abstraction, Interaction, and Insight.” Pragmatics & Cognition 22(2):264–283.