The promise of generative AI technologies is seductive to product leaders: frictionless research in which...
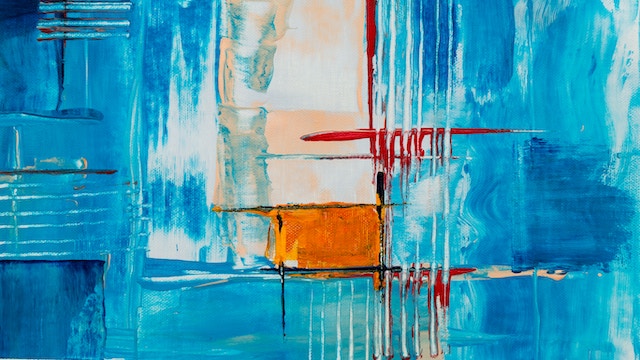
The promise of generative AI technologies is seductive to product leaders: frictionless research in which...
Case Study—This case study presents ethnographic work in the midst of two fields of technological innovation: automated vehicles (AV) and virtual reality (VR). It showcases the work of three MSc. Techno-Anthropology students and their collaboration with the EU H2020 project ‘interACT’, sharing the...
This paper provides a theoretical alternative to the prevailing perception of machine learning as synonymous with...
Case Study—We report on a two-year project focused on the design and development of data analytics to support the cloud services division of a global IT company. While the business press proclaims the potential for enterprise analytics to transform organizations and make them ‘smarter’ and more...
The wide-spread deployment of machine learning tools within healthcare is on the horizon. However, the hype around “AI” tends to divert attention toward the spectacular, and away from the more mundane and ground-level aspects of new technologies that shape technological adoption and integration....
Case Study—This case-study details how a team of anthropologists and a team of data scientists sought to help a Middle Eastern theme park make use of their big data platform to measure ‘the good customer experience’. Ethnographic research within the theme park revealed that visitors yearned to...
Few professions appear more at odds, at least on the surface, than ethnography and data science. The first deals in qualitative “truths,” gleaned by human researchers, based on careful, deep observation of only a small number of human subjects, typically. The latter deals in quantitative “truths,”...
Case Study—Declining clinician engagement, increasing rates of burnout, and stagnant patient and family experience scores have led hospital leadership at Seattle Children's Hospital to submit requests to a data scientist and an anthropologist to identify key themes of survey comments and provide...
The focus of this paper is to investigate deep learning algorithm development in an early stage start-up in which edges of knowledge formation and organizational formation were unsettled and contested. We use a debate by anthropologists Clifford Geertz and Claude Levi-Strauss to examine these...
Case Study—How can we build fairness into automated systems, and what evidence is needed to do so? Recently, Airbnb grappled with this question to brainstorm ways to re-envision the way hosts review guests who stay with them. Reviews are key to how Airbnb builds trust between strangers. In 2018 we...