The promise of generative AI technologies is seductive to product leaders: frictionless research in which synthetic data can be both generated and analysed via a simple end-to-end UI, enabling teams to speed up research timelines and reduce costs. However, our evidence suggests we should be sceptical of these maximalist claims. Over the last 18 months our combined team of NLP data scientists and ethnographers has conducted a series of experiments to explore, assess and define the value of LLM-driven research techniques. First we explore this value pragmatically, as new tools for sensemaking; and second, epistemologically, as we unpack their broader implications for ethnography. We demonstrate how ethnography can usefully “ground” LLMs in two “complex” worlds: that of the user and that of the organisation. We argue the future of research is not automation, but more collaboration between ethnographers and data scientists, as they better integrate their tools and ways of knowing. Keywords: sensemaking, large language models, natural language processing, generative AI, cynefin framework
INTRODUCTION
In technology companies, ethnography has often had an uneasy, even subservient relationship to quantitative and data science research. It remains the case that “large-scale patterns drive decision making” (Levine, 2019). By comparison ethnographic research can be regarded as small-scale and inconclusive. Many teams perceive research as a necessary evil that “slows down” (Belt, 2019) the agile approach to product development, the inconvenient human factor in an otherwise frictionless loop.
A new wave of tools are being enthusiastically adopted to circumvent traditional user research and speed up product iteration. A / B testing capabilities are increasingly cheap, personalised and rapid, enabling product teams to jump ahead with their hypotheses and collect actual usage data rather than wait for a user research process to deliver. Research is squeezed in the process, often constrained by its tactical role within product development, and then ostracised for its inability to deliver timely results.
It is within this context that Large Language Models (LLM) have arrived. In recent years a range of natural language processing (NLP)-enabled tools have targeted the ethnographic market, either claiming to speed up analysis (e.g. Reduct) or scale up cultural datasets to increase decision making confidence (e.g. Motivbase).
In the last six months, the accelerating capabilities of generative models such as GPT-4 have made these assertions bolder, with some experiments producing results that call into question the need to conduct primary research in the first place (Argyl, 2022). Services like Synthetic Users leverage the latest models to enable researchers (and non-researchers) to define the user they want to interview, and then generate simulated responses to any question they care to pose.
The promise of these technologies is seductive to product leaders: frictionless research in which synthetic data can be both generated and analysed via a simple end-to-end UI, enabling teams to speed up timelines and reduce costs. As such, NLP poses a potentially existential threat to ethnographers working in corporate settings.
This paper argues there is no existential threat to ethnography (or primary qualitative research more generally). Through a series of experiments we demonstrate that the value of ethnography is not replaced by these new technologies, but rather augmented and clarified by them. The ethnographic skill set remains vital because it is capable of exploring domains that are, by definition, not comprehensible to these new tools. And we show why this is true for sensemaking across both organisational and user settings.
The paper shows how ethnography’s value can be elevated by NLP. As the technology automates time consuming work, the ethnographer is freed to exercise their unique capacity for exploring complex domains. Exploring complexity, we conclude, is a highly desirable skill set in a world dominated by LLMs.
The History of NLP in the Social Sciences
Before we explore the implications for ethnography specifically, first we need to situate the history of these new capabilities in the wider space of social sciences and the problems they seek to address within them.
Though the current focus of the paper is on generative AI and its potential applications in ethnographic research, we need to understand the technology that sits behind it. NLP plays a vital role in providing the technical backbone for the text generation capabilities of the recent LLM tools.
Natural language processing (NLP) is the interdisciplinary field that seeks to analyse written and spoken language using computational approaches. It is especially useful when applied at scale to large volumes of data, the analysis of which is otherwise infeasible without considerable human effort and costs. In its early stages, NLP was predominantly used as a means for content classification based on rule-based heuristics and carefully curated lists of terms (LIWC, black lists etc.) which are then matched against the document input. With the rise of deep learning and higher computing power in the last 5 years, the field has been increasingly growing with industry-wide applications ranging from sentiment analysis of product reviews, machine translation, detection of online offensive language to many other domain-specific use cases.
NLP has proved useful in the social sciences. NLP tools have been used to support the analysis of open-ended questions in surveys (Xu et al., 2022; Meidinger and Aßenmacher, 2021) due to its potential to mitigate the trade-off between obtaining rich data and manually coding many responses (Beeferman and Gillani, 2023). It has also been used to quantify inherent bias present in datasets. This is done by investigating word co-occurrences at scale which can be systematically assessed via word embeddings. Given the large amounts of data necessary to construct these, social scientists have analysed the resulting word representations as reflections of the cultural assumptions and social biases in the data (Lauretig, 2019; Nelson, 2021). Finally, NLP has long been linked to the task of modelling mental models as suggested by Plantin (1987). The rise of online forums and social media platforms allows the online representation of large communities of interest, thus providing validation to smaller in-person studies. In fact, recent works have shown the value of NLP to uncover community-wide views (Strzalkowski et al., 2020; Kaur et al., 2022).
Step-change to Large Language Models
Large language models have revolutionised the field of NLP and encouraged the adoption of data science-centric approaches in most fields and industries. One driving factor behind the acceptance of these tools is their accessibility to a non-data science audience. Examples of this include the simple chat interface provided by ChatGPT (OpenAI), and the integration of LLMs into the official Bing search engine for more tailored search results. This along with wider context windows and the recent capability to analyse multimodal input have been instrumental in the adoption of LLMs.
The success of LLMs is partly owed to the emergence of contextual word embeddings. These are dynamic vector representations of a word based on its meaning given a surrounding context. For example, the word “right” in the sentence “The justification is right” is different from “He dislocated his right arm” and should thus be encoded by different numerical representations so that the LLM can “understand” the distinction between the two. The progress from static to dynamic representations as well as the availability of vast online training data and computing resources have enabled the creation of large language models capable of generating fluent output.
Since their introduction, LLMs have been shown to consistently define new state-of-the-art performance across many NLP tasks that require natural language understanding (Barbieri et al., 2020) such as reading comprehension or question-answering where “superhuman” performance is achieved (Bowman, 2023). This step change in capability leads to questions about the importance of human involvement in automated ecosystems where LLMs already promise faster delivery and better results than human annotators. However, AI researchers such as Tedeschi et al. (2023) bring attention to the need to critically evaluate these models using reliable metrics and realistic settings before deploying them as a replacement to human judges.
As LLMs were gradually adopted as conversational agents used for brainstorming, acting as a “user’s creative and helpful collaborator” (BARD), academics began investigating the potential of LLMs’ cognitive performance. In essence, this involves assessing the capability of an LLM as one would a human, for example via evaluation criteria adopted from the field of psychology: creative ability (Stevenson et al., 2022), reasoning (Binz and Schulz, 2022), personality testing (Miotto et al., 2022). In particular, Miotto et al. investigate GPT-3 by qualitatively assessing 3 dimensions (personality type, human values, and demographic characteristics) using established self-report tests such as the Human Value Scale (Schwartz, 2003) employed by the European Social Survey. The aim of the study is to uncover and understand the LLM personas created by varying the “temperature” parameter within the model, while keeping all others at their default value. Temperature controls the predictability of the generated text with values ranging from 0 to 1 where 0 ensures a nearly deterministic response and 1 induces significant randomness. They find that varying the temperature leads to model fluctuations across all the afore-mentioned dimensions. For instance, when asked what gender and age it identifies as in the prompt, the default GPT-3 identifies as a female entity in late twenties and increasing the temperature leads to a higher proportion of male gender responses and lower age. Similarly, other dimensions are impacted with more extreme tendencies exhibited the higher the temperature.
These experiments and the emergence of improved LLMs (like GPT-4) facilitated the potential creation of “synthetic users.” Companies such as Feedback by AI and Synthetic Users deliver outputs to specific prompts that mimic human feedback. Synthetic data can be instantly generated by imposing specific criteria including profession, marital status and personality traits in accordance to the population a study requires. These platforms promise it is possible to glean user insights about your product or service while foregoing the costs and time needed for recruiting and interviewing real people.
Existing studies by Google (Weidinger et al., 2021) warn against the potential risks involved with LLM downstream applications. Word embeddings, at the core of all LLM operations, have been repeatedly confirmed to exhibit gender bias and lead to harmful representations for both BERT (Jentzsch and Turan, 2022; Touileb and Nozza, 2022) and GPT-3 (Lucy and Bamman, 2021). Moreover, recent work done by Kantar (2023) urges against the perceived value of synthetic samples and shows that experiments substituting human panellists lack insights into population subgroups or specific topics, and exhibit strong positive bias. This along with data privacy concerns and the lack of transparency of the data used to train these models are reasons to apply caution in LLM large-scale usage to avoid propagating social stereotypes and unfair discrimination.
Assessing the Value of NLP for Ethnographic Sensemaking
The potential for these technologies is wide ranging across the social sciences. But what are the implications for ethnographic and qualitative enquiry specifically? Stripe Partners’ data science and ethnography teams have been collaborating on a series of experiments focused on applying NLP to sensemaking, a key aspect of the ethnographic research process.
We define Sensemaking according to Organisational Studies scholar Karl Weick (Weick, 1995). For Weick, Sensemaking is “the negotiation and creation of meaning, or understanding, or the construction of a coherent account of the world” (MacNamara, 2015). Sensemaking is a critical aspect of ethnographic research in corporate settings. It is the process by which we (and our stakeholders) make sense of both the subject we have been commissioned to understand, and the organisational endpoint where insights and recommendations will land, to arrive at a shared path forward. Sensemaking is “successful” when it is (a) true to the data (b) meaningful to the people for whom understanding is important, and (c) leads to the successful accomplishment of intended outcomes. This is what expands sensemaking beyond “analysis”. Analysis focuses on the correct interpretation of data, but discounts the social dynamics of meaning creation, and, by extension, organisational impact.
For Weick, Sensemaking is a highly contextual, contingent process that can pivot on seemingly trivial moments. “Students of sensemaking understand that the order in organizational life comes just as much from the subtle, the small, the relational, the oral, the particular, and the momentary as it does from the conspicuous, the large, the substantive, the written, the general, and the sustained. To work with the idea of sensemaking is to appreciate that smallness does not equate with insignificance. Small structures and short moments can have large consequences.” (Weick, 1995)
Sensemaking is a critical aspect of the ethnographic research process from three perspectives. First, our research expertise lies in observing and capturing “the subtle, the small, the relational, the oral”. These phenomena are rarely represented in existing data and documentation, and are usually illegible to corporate systems. Businesses recognise the value of this knowledge to improve decision making, which often makes it the focus and rationale of ethnographic study.
Second, because the questions ethnographers explore are complex, and the insights difficult to “prove”, successful practitioners engage and enrol stakeholders in their research process, so that the resulting findings are meaningful and “embodied” by the people who will enact them (Roberts and Hoy, 2015). By engaging in research as a social process of sensemaking rather than assuming the facts will “speak for themselves”, ethnographers ensure their work has influence and impact.
Third, as Weick argues, tacit social codes and dynamics are just as significant within organisations as they are outside of them, and thus it is best practice for ethnographers to study the organisational context they are seeking to impact as part of the sensemaking process. What they learn helps them to filter and shape their work to maximise its utility and influence within that specific organisation.
It is within these sensemaking contexts that the contribution of NLP will be judged.
NLP EXPERIMENTS IN SENSEMAKING
Over the last 18 months Stripe Partners, an innovation consultancy based in London, hired two expert NLP practitioners to join its data science practice. At Stripe Partners, our mission is to create a new discipline at the intersection of social science, data science, and design that delivers more effective product innovation for technology-led clients. NLP was identified as a practice within Data Science that is well placed to combine with and complement the more qualitative forms of research practised by ethnographers in the business, given its focus on language and, by implication, human culture and systems.
To explore the possibilities, we ran 15 experiments utilising LLMs across multiple client projects, using a variety of NLP techniques.
Here, we will share three specific experiments across a spectrum of ambition. The first experiment seeks to understand the value of NLP as a discrete analytical tool for the ethnographer. In the second we explore how NLP could be used to increase confidence in qualitative data as part of a broader ethnographic sensemaking process. Finally, the third experiment evaluates the possibility of using NLP to “replace” the ethnographer within a closed loop, automated sensemaking system.
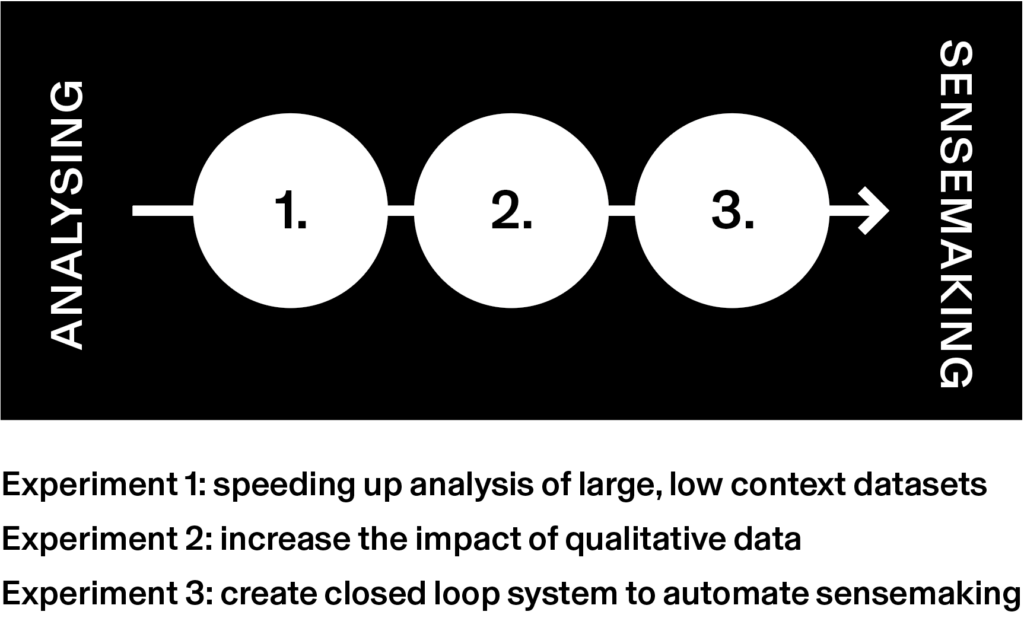
Experiment 1: Speeding up Analysis of Large, Low-Context Datasets
The Experiment
The client we were working with was a healthcare company that asked us to provide an overview of the patient experience regarding existing medical treatments for a specified condition. Following the success of previous interdisciplinary studies which used online data to gauge patient attitudes (Brezulianu et al., 2022) and reactions to new treatments (Yadav et al., 2018), we identified a healthcare dataset of hundreds of posts and users as candidates for analysis. In our first experiment, we showcase the potential of LLMs to speed up analysis of large amounts of data.
Often in qualitative studies researchers are confronted with large datasets of low context data, such as open-ended survey questions or anonymous postings on online forums. The process of manually analysing these corpora is time consuming, and is often conducted by more junior researchers. Thanks to its conceptual simplicity (it classifies text as negative, neutral or positive), we propose sentiment analysis as a high-level tool to automatically synthesise such data. As its outputs are immediately interpretable, this method can be a support to social scientists for dealing with low-context datasets.
We used both BERT (Google) and GPT-3.5-turbo (OpenAI) as our testing baselines and discussed the need for human-in-the-loop evaluation in both LLMs. We observe that despite the improved performance of GPT over the older BERT model, both default models exhibited shortcomings which can be overcome through critical human evaluation via error analysis and few-shot tuning.
Error analysis was carried out to identify data patterns where the sentiment analysis model was consistently incorrect. For example, we observe that BERT overly classified instances as negative when patients self-disclose their condition, whereas GPT failed to detect negative instances when painful secondary symptoms or targeted opinions about medicine are discussed. We note that this evaluation can lend itself to any participant within the team since the task does not require any specialised knowledge to assess model mistakes. Once a set of representative examples is collated, this can be used for refining model judgement through fine-tuning.
Few-shot training is a fine-tuning process of re-training an off-the-shelf model for a specific task or a specific domain by “showing it” a small sample of annotated human judgements. While for older models such as BERT, fine-tuning remains a data science dominated approach, recent LLMs allow for a more collaborative interaction between data scientists and ethnographic researchers enabled by prompting techniques via the conversation interface.
As recent work has shown, the amount of information within a prompt and its style have a significant impact on the LLM performance (Shen et al., 2023). Chain-of-thought reasoning (Wei et al., 2023) is an emerging trend that equips a prompt with a set of intermediary steps which decompose a complex task, similar to a human thought process. We have experimented with varying levels of prompt complexity, and concluded that the prompts exhibiting chain-of-thought reasoning and including task demonstrations yielded the best accuracy. Our final prompt (See Table 1) for Sentiment Analysis in the healthcare study included a brief note on the data description, a rigorous task formulation where each possible label was defined with reasoning guidelines and finally, reasoning-enhanced examples. The construction of these components is an example of how ethnographic researchers and data scientists can efficiently collaborate to understand large amounts of data.
Table 1. Prompt Example for the task of Sentiment Analysis in Healthcare and Evaluation of prompt complexity
Prompt Example | |
Data Description | You will be shown a Sentence extracted from a microblog thread discussing the [condition]. |
Task Formulation | Please classify the Sentence as negative, neutral or positive. |
Reasoning Guidelines | Negative: The Sentence contains information about painful user experiences OR declining health OR negative opinions about products.Positive: The Sentence contains information about happy user experiences OR improved health OR positive opinions about products.Neutral: The Sentence does not contain any of the above. |
Example with Reasoning | Sentence: It’s been my experience that if I’m feeling a bleed starting then that means [treatment] alone won’t stop it.Justification: negative opinion about product Answer: negative |
BEFORE: Tested Example with Simple Prompt (Data description + Task formulation) Sentence: That might be why [treatment] doesn’t completely do it for me. Answer: neutral | |
AFTER: Tested Example with Complex Prompt (Data description + Task formulation + Reasoning Guidelines + Examples) Sentence: That might be why [treatment] doesn’t completely do it for me.Justification: negative opinion about product. Answer: negative |
In Table 1, we include tested examples of before and after prompt enhancement. Based on the simple prompt, the model made wrong assessments of medicine-targeted sentiment: [treatment] is negatively discussed in the context “That might be why [treatment] doesn’t completely do it for me.”, but GPT-3.5 incorrectly classifies this as neutral. We observe that this error was rectified when the model was trained on a complex prompt. With the help of manually coded examples (known as “few shot prompting”), GPT-3.5 now correctly evaluated the testing instance and assigned the correct sentiment (“negative”) and appropriate justification (“negative opinion about product”) which helps improve model transparency.
Discussion: NLP Provides Researchers with a Useful, Standalone Analysis Tool
Experiment 1 demonstrates that with the correct prompts, NLP is an excellent tool for speeding up the analysis of low context datasets within the context of a wider sensemaking process such as open ended surveys, social media discourse or remote interview / diary transcripts.
The accessibility of tools such as ChatGPT, Bing, Claude and Google Bard enables researchers with limited training in computer or data science to fast track discrete analytical tasks when they are confronted with large qualitative datasets. This has significant benefits for ethnographers under pressure to “speed up”. If used judiciously for specific tasks within a wider process, NLP can definitely save time.
In this experiment we utilised sentiment analysis, but there are other forms of analysis (see Table 2) that we have experimented with and can provide value for discrete tasks within the context of a wider sensemaking process. Researchers should consider utilising these tools when the following analytical tasks are relevant.
However, it is vital to prompt these tasks correctly. Our experiment taught us it is critical for researchers to “train” the models with (a) precise definitions of any categories they want the analysis to incorporate and (b) several examples of the correct analysis. This is called “few shot prompting”, and dramatically increases the quality of the analysis.
The best way to achieve this successfully is by conducting manual analysis of a limited subset of the data yourself (4-5 snippets is usually sufficient). Where possible, focus this manual analysis on esoteric edge cases, where definitions and categorizations are likely to be contested and / or are driven by specific requirements of the project. This may take some trial and error. When the model has generated its response, manually analyse a subset of the response to ensure it meets quality expectations. If it doesn’t then rewrite the prompt with more instances of manual analysis that are focused on correcting the errors observed and/or making further clarifications of definitions.
Table 2. Overview of NLP analysis types, their recommended usage and example use cases
Analysis Type | Useful to... | Example Use Case |
Sentiment Analysis | …determine the sentiment or emotional tone of a piece of text, such as positive, negative, or neutral. It aids understanding of the overall opinion or attitude expressed in the text. | Analysing public forum posts to assess the attitudes towards a new product(see example above) |
Topic Modeling | …discover abstract topics within a collection of documents or text corpus. It helps identify the main themes or subjects discussed in the text without prior knowledge of the topics. | Analysing online reviews to capture the main pain points experienced when using a new service |
Text Classification | …assign predefined categories or labels to text documents based on their content. It is useful for tasks such as spam detection, sentiment classification, and document categorization. | Analysing the prevalence of user needs identified through interviews within wider social media discourse |
Language Translation | …translate text from one language to another while preserving the meaning. It enables communication and understanding between individuals who speak different languages. | Translating foreign language interview transcripts into legible language |
Text Summarization | …generate concise and coherent summaries of longer texts, capturing the main ideas and important details. It helps in digesting large amounts of information quickly and efficiently. | Creating short summaries of interview transcripts highlighting key topics covered |
Semantic Search | …retrieves a list of evidence semantically matching a specified query from a large body of documents. It improves standard search accuracy as it does not rely on strict word overlap. | Finding evidence within a set of articles to increase confidence in an emerging finding |
The experiment discussed was conducted using Google BERT and ChatGPT 3.5 Turbo. Our expectation is that newer models such as GPT 4 will reduce the requirement for few shot prompting as their overall understanding of language improves. However, for reasons we will expand on next, we believe that intelligent prompting and manual checking of analysed results will remain critical tasks for the foreseeable future, as part of a broader sensemaking process.
Experiment 2: Increase the Impact of Qualitative Data
The Experiment
Our second experiment explored how LLMs can be used to increase confidence in the ethnographic sensemaking process through an open-ended enquiry. Ethnographic studies impacted by strict recruitment criteria and /or small samples can especially benefit from analysing a wider online community using NLP methods.
In this instance our research goal was to understand the advantages and disadvantages introduced by different medicine types to patients diagnosed with a specified rare condition affecting less than 0.0001% of the population worldwide. In our case, the project focused on investigating the experiences of these patients in a specified country, which significantly narrows the pool of candidates. We conducted interviews with a dozen subjects, and then enriched our analysis by incorporating hundreds of online patient conversations on the topic. Particularly, we follow the success of other works employing social media platforms for medical applications (Park et al., 2018) and construct an extended data sample from the subreddit “r/[condition]”, a forum used by patients from across the globe to share their experiences about this condition. The data is collected using the API of the Reddit microblogging platform.
This approach makes use of topic modelling, an NLP clustering technique that groups semantically similar content, i.e. posts which discuss the same topic. We employed BERTopic (Grootendorst, 2022), a topic modelling algorithm based on large language model BERT. An LLM-enriched approach such as BERTopic can uncover high-level connections between similar concepts (e.g. “syringe” and “injection”) by making use of its external knowledge as opposed to just relying on word overlap.
Similar to sentiment analysis, the application of topic modelling must be appropriately tuned to each dataset. This includes specifying model parameters such as the number of resulting topics or the maximum size of topics. This is often an iterative process which requires careful inspection of the topics in each round. Additional human analysis can be conducted to place misclassified posts in correct topics, a process inspired by computational grounded theory (Nelson, 2020). The last step of the topic modelling focuses on summarising the resulting topical groups of posts. We include examples of extracted topics in Table 3.
Unlike Experiment 1, this method’s output needs to be grounded with insights from the ethnographic project to be useful. Ultimately, some topics produced by BERTopic are not useful, while some are valuable to complement or augment the knowledge gained from interviews. Assessment of a topic’s potential is determined by its ability to answer the research questions posed by the client (i.e. advantages and disadvantages to different medicine types) and its suitability to the target users the client needs (i.e. patients from a specified country).
Table 3. Examples of topics generated by BERTopic topic modelling tool in Healthcare. Each topic is evaluated with respect to its usefulness for the ethnographic studies (evaluation) which is then supported by the analysis.
# | Topic Description | Evaluation | Analysis |
1 | The topic focuses on discussion about negative aspects regarding modes of administration: 1. Subcatenous administration: Some users disclose accounts of muscle pain and skin irritation around the injection site (belly, arm). Other users express feelings of nervousness for subcutaneous administration in the belly. 2. Vein administration: Accounts of painful /broken veins are shared. | Useful for complementing ethnographic studies | This topic came up in the qualitative findings based on second-hand accounts (nurses) of the symptom, but did not surface directly in interviews, so was discounted until its importance was highlighted through the NLP analysis. |
2 | The topic focuses on issues around trusting how and whether a specific medicine works; these views are expressed by both users and potential users. | Useful for validating ethnographic studies | Both qualitative (interviews) and quantitative data (hundreds of Reddit posts) reveal the need for trust that a medicine works. |
3 | The topic covers the experiences of patients from different demographics around the world with respect to medicine access. | Potentially useful | Medicine access is highly subjective to the country the user patient is in. The topic was ultimately not useful to ethnographers as it did not target the patients from the country specified by the client. |
The useful topics generated by BERTopic have a dual purpose: (1) identify emerging information which has not been previously surfaced in the field interviews (complement) and (2) provide confidence supported by big data to already known results (validate). An example of a useful topic is Topic 1 in Table 3: our study on the Reddit corpus uncovered that the administration of a type of medicine leads to skin irritation for some patients; this aspect was not immediately visible in the interviews conducted by the ethnography researchers. Also in Table 3, we find Topic 3 as an example of a topic uncovered by NLP findings which is ultimately evaluated as useless by the qualitative team: while addressing an important aspect of the patient experience (medicine access), this aspect is highly dependent on the medical system in each country; consequently, the topic does not bring any value because it does not target the population specified by the client.
Discussion: NLP Can Increase Confidence in Qualitative Work, but Always Requires “Grounding”
If Experiment 1 taught us that LLMs require prompting to produce high quality analysis, a more fundamental challenge is exposed in Experiment 2: the “symbol grounding problem” (Harnard, 1990). To be meaningful and useful, language must be deployed within a specific context. The symbol grounding problem points out the fact that large language models operate in closed, self-referential systems that do not account for shifting human contexts. As Bender and Koller explain, “language is used for communication about the speakers’ actual (physical, social, and mental) world, and so the reasoning behind producing meaningful responses must connect the meanings of perceived inputs to information about that world.” (Bender and Koller, 2020). Because these statistical models have become deracinated from the world that produced the data to train them, this process of “grounding” must take place to generate meaningful, useful outputs.
In Experiment 1, providing examples of correct analysis drastically improved the quality of the automated LLM analysis. Because the discrete task was to correctly categorise the sentiment of different sentences, the quality of the analysis could be assessed without recourse to the specific requirements and context of the study. To put it another way, wrongly categorising a statement as “neutral” when it was, in fact, “negative” requires only a good grasp of (English) language and, at times, an understanding of the linguistic vagaries of online healthcare discourse. As such, it was possible to objectively assess and improve the quality of analysis within the closed system of language.
In Experiment 2, however, it was not sufficient to increase the model’s competence with language. Here, the intent was not to speed up analysis, but to increase confidence in the ethnographic work by expanding the surface area of data to incorporate public online forums. The BERT-driven topic modelling of these forums successfully identified multiple themes relating to the treatment of study that were not identified in the qualitative work, and it could tell us which themes were most common. However, presenting our client with the most popular themes was of limited value: many were esoteric and/or irrelevant to the requirements of the study.
This begs the question, how did we know which topics are esoteric or irrelevant? First, from our ethnographic work we have a rich, behavioural, up to date understanding of the condition and treatment, which we can use to assess what themes are coherent with this more holistic understanding of the patient experience, and which are anomalous. Second, we have a rich understanding of the organisational context. We understand the specific sensibilities of each stakeholder; the politics of how decisions are made; the wider corporate strategy and context. These are the “small” (Weick, 2005), nuanced contextual layers that are entirely invisible to an LLM, but are critical to producing work of value.
In this experiment, knowledge of these two contexts enabled us to identify topics that either (a) validated existing insights from the ethnographic work or (b) helped us to identify complementary, parallel insights. Leveraging knowledge of these domains maximises the value of NLP, strengthening ethnographic work by either validating emerging insights or highlighting lateral, complementary insights.
The NLP analysis therefore had the effect of increasing client confidence in the project, augmenting the data gathered through the ethnography. This experiment would not have been successful, or even possible, without the involvement of the researcher who triangulated the range of topics identified by the LLM to produce work that is relevant and impactful. In this sense these LLM tools can augment and validate the work of the researcher, but cannot replace them.
Experiment 3: Create Closed Loop System to Automate Sensemaking
The Experiment
Our client was a content platform that matched billions of users with billions of pieces of video content. The team we were working with was focused on improving video recommendations for would-be travelers exploring potential holiday destinations. The current recommendation system was judged to be poor for travelers using videos to inform their planning. In this third experiment we wanted to see if it was possible to use NLP to identify the underlying needs that different videos addressed, and then use that insight to further improve the recommendation system.
The research team curated a corpus of relevant videos spanning different formats, styles, creators and subjects. This is then used to collect a larger text-based dataset comprising 40k comments posted about these videos which formed the basis for the NLP analysis. We focused on comments (versus the video content itself) because they are user-generated, and therefore the best available qualitative signal of value from a user perspective.
This experiment also used approaches such as sentiment analysis and topic modelling which are ideal for initial exploration of the data (Bottom-Up), but additionally introduces new tools such as semantic search better suited for top-down analysis. A Top-Down approach starts with a concept (in our case a content need) and allows for a narrower search of the data by retrieving comments which reflect the concept.
We find that while topic modelling across the set of comments in our corpus of videos reveals the nature of conversations being generated (See Table 4 for some examples of topics), these topics are too general and do not in themselves identify specific user needs. It was by interviewing users qualitatively who had also consumed the videos that we could identify what to “look for” in the comments. Interviewing future and past travellers allowed us to uncover 11 criteria, called travel needs, that an ideal travelling video should satisfy based on its content and creator. Once the travel needs were defined, they were used to shift the focus from a bottom-up exploration to a guided Top-Down process. For example, Topic 3 in Table 4 discusses recommendations for relaxation venues, often posed as questions. Insights from the interviews revealed affordability as an important user need for assessing a potential destination /experience. In light of this, Topic 3 now proves to be representative for the Affordable need as it contains comments asking for practical advice, a key notion for this criterion. Table 5 includes a few illustrative comments uncovered by inquiry models and semantic search; the comments discuss aspects such as accommodation, food and activities which are important aspects a video needs to cover in order to be ‘affordable’ to potential travellers. Following a similar approach, we find that 8 out of a total of 11 travel needs discovered in the qualitative study can be partially predicted using the video comments.
Table 4. Examples of topics generated by topic modelling from Video Comments which are accompanied by high-level analysis on their potential use.
Bottom-Up Approach | ||
# | Topic Description | Analysis |
1 | Discussion focuses around food and recommended cuisines (Cuban, Jamaican) and dishes (sandwich, tacos). | The topic uncovers food as an important aspect to potential travellers which can be used to draw inspiration. |
2 | Discussion includes statements about how much people love or like the video, vlog or channel (and aspects in it like editing). | The topic captures the emotional connection inspired by the video and can be used to bridge the role of comments to other potentially useful non-linguistic features such as video style or creator. |
3 | Discussion focuses on relaxation venues: clubs, parties, pools, lounges. Comments are often posed in question form such as users asking about recommendations or asking for practical advice such as the budget needed. | The topic caters to the research stage of the user’s planning journey because it generates many questions within its comments. |
Table 5. Example of comments extracted from comment video sections which are representative for the predefined ‘Affordable’ travel need.
Affordable = “I need to be able to easily find out about a destination / experience to assess how feasible it is for me, and how to make it happen” | |
Video Comments | Relevant Aspects |
Hey, how much were the yacht and the jet skis? | Activity |
What was the name of the airbnb you stayed at and how much did it cost? | Accommodation |
Tell us how $$ much $$ each taco plate costs. | Food |
Despite our efforts, the predictive power of comments is limited. First, not all travel needs are legible, and, second, additional signals must be identified for the recommendation system to correctly categorise a video. To mitigate such cases, in the second part of the experiment we constructed a database of complementary signals that can be extracted from the platform’s metadata using their API. An example of a travel need that cannot be predicted is Expert defined as ‘‘I need to see content from people who are experts in the subject so I can trust what I’m watching is the best’’. As this travel need is best described in terms of the video creator, nonlinguistic metadata attributes such as the verified status of the creator and the number of user likes are a better validation criteria than comments. Even for travel needs that can be partially predicted by comments, we recommend strengthening the confidence of our evaluation by considering attributes beyond comments. For instance, affordability can be tested against whether the video description provides links to the places discussed within the video.
Discussion: Translating between Complex and Complicated Problems
Experiment 2 introduced us to the fundamental limitation of NLP for sensemaking, namely the “symbol grounding problem.” Experiment 3 further elaborates the implications of the symbol grounding problem when attempting to operationalise and automate NLP to create a closed loop system to improve the performance of a recommendation system. In the first part of the experiment, NLP was deployed to identify signals in user comments that reveal why a particular video resonated with its audience. The resulting topics identified were superficial because the LLM did not have any insight into the deeper motivations of people who found value in the videos.
The complementary in-depth interviews enabled researchers to probe deeper and identify the underlying travel needs that specific videos addressed for viewers.This data was unavailable to the LLM because it has not been surfaced and captured before. Once these more nuanced, situated motivations– or “needs”– were identified through interviews with research participants, it was possible to re-categorise the video comments around the identified needs. Once it “knew” the needs through our prompting, the LLM could identify 8 /11 needs in the comments.This experiment demonstrated the value of primary qualitative research, to discover and interpret nuanced, emergent patterns in specific contexts that are not included or visible to the underlying models powering LLMs.
In the second part of the experiment we explored whether it was possible to automatically predict whether a specific video was delivering against the pre-defined needs using only the comments as an input. Here, we discovered that while the comments do provide sufficient signal for a minority of needs, it was critical to add additional signals, including language-based signals (e.g., transcript, title) as well as non-linguistic metadata (e.g., creator verification, upload date, view count, subscription numbers) to more confidently predict that the video was addressing a particular need. This second dimension raises the question of how much “signal” language alone provides, and highlights the dimension of ethnography that extends beyond language to encompass the observation and analysis of complex, non-linguistic phenomena through “thick description” (Geertz, 1973). More broadly, it points to the increasing complexity of the consumer environment, and the requirement for ethnographers to be more than “the voice of the user” and critically engage with the deeper socio-technical systems that shape behaviour (Anderson et al., 2012).
David Snowden’s Cynefin Framework (Snowden and Boone, 2007) usefully distinguishes between the growing importance of solving “complex” problems, in contrast to the more predictable “complicated” problems that are already legible to existing systems. Complicated problems can be addressed through expert knowledge and rules, what Snowden calls “known knowns.” Complex problems involve unknown unknowns and are characterized by emergent conditions, non-linear dynamics, and unpredictable human behavior.
“Complicated problems can be hard to solve, but they are addressable with rules and recipes, like the algorithms that place ads on your Twitter feed. They also can be resolved with systems and processes, like the hierarchical structure that most companies use to command and control employees. The solutions to complicated problems don’t work as well with complex problems, however. Complex problems involve too many unknowns and too many interrelated factors to reduce to rules and processes.”
(Kinni, 2017)
In this sense, matching user needs to relevant travel videos is a complicated problem when a data scientist or engineer already knows what the viewers’ needs are. But to identify what those needs are in the first place, that’s complex.
Experiment 3 therefore further highlights the extent to which machine understanding of contingent, specific, human domains is limited. GPT-4 may be trained on 60 billion parameters, but that is still miniscule compared to the dynamic, emergent, multi-faceted dimensions of human culture and behaviour. The role of ethnographers is to explore these complex problems and attempt to translate them into complicated ones. In this case, that was making the underlying value of different travel videos legible to a recommendation system.
Before, our client’s question of what needs do travel videos address was a complex question; now we have uncovered and mapped those needs to concrete, machine-legible attributes, both linguistic and nonlinguistic. We have translated the problem into the complicated domain (that is until culture evolves sufficiently to make this illegible again). Once in the complicated domain and solvable with pre-existing data, it is possible for engineers to translate these into repeatable processes that can be automated by systems like the recommendation engine in our example.
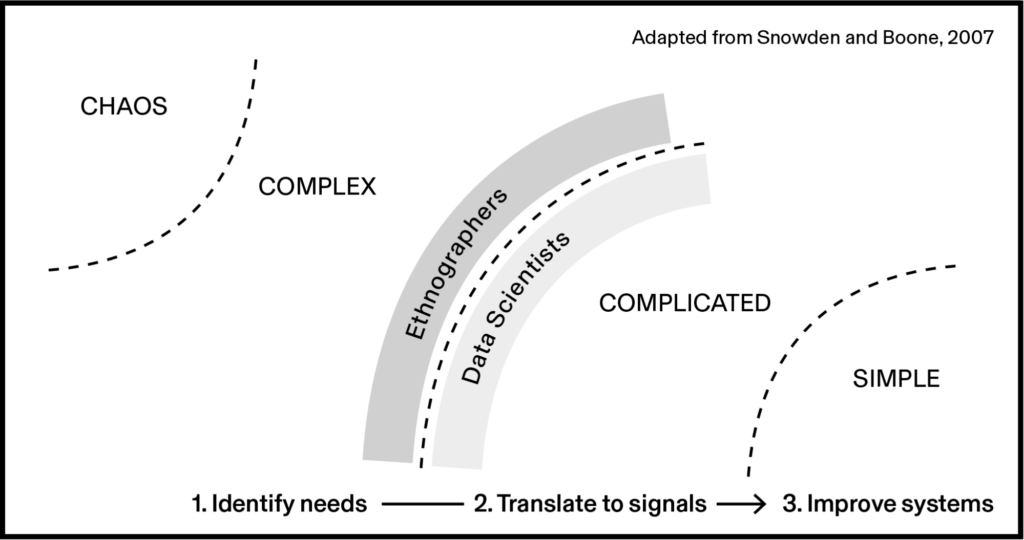
These models are incapable of “sensemaking” when it is critical to account for these “complex” domains because they are, by definition, not yet legible to them.
Ethnographers, in contrast, are well placed to explore the uncharted fields that are not represented within structured or unstructured datasets. Working with data scientists, ethnographers should seek to translate insights from these complex domains into complicated domains, by identifying existing or new signals that are legible to machine systems (including LLMs). Focusing on this intersection between complex and complicated showcases ethnography’s strengths, and helps ethnographers clearly articulate the value of ethnography within their organizations.
CONCLUSION: ETHNOGRAPHY AND LLMS TODAY AND TOMORROW
Language, divorced from humans, is a closed, self-referential system. Large Language Models scale this system by training it on a vast corpus of data. They use statistics to predict the most likely linguistic response to a given query. Because they use pre-existing datasets to solve queries they are, by definition, complicated systems.
LLMs are optimised through human reinforcement learning. In practical terms this means people are paid to review possible responses to a given query and tell the machine which option makes most sense. To determine what “makes sense” these human assessors implicitly draw on their nuanced understanding of culture, ethics and expertise specific to their personal contexts. They draw from complex, human domains.
It is simply not feasible for LLMs, via human reinforcement learning, to always, already scale to every evolving complex context. This is why the ethnographer is in a resilient position: there is always uncharted territory to explore. And more importantly, businesses will always be interested in complex domains because they are a source of competitive advantage. In short, there is a strong motivation to map unchartered territory first and integrate it into your operating model before your competitor does.
Rather than replace ethnographers, LLMs can complement and accelerate their work as they explore complexity. As our experiments demonstrated, when deployed as an analytical tool by researchers and data scientists embedded in a wider sensemaking process, NLP can offer significant value.
We learned from our first experiment that when ethnographers are faced with large, deracinated healthcare datasets to analyse, LLMs can speed up repetitive analytical work through a range of approaches. But only if given sufficient context and precise categories through intelligent “few shot” prompting.
In the second experiment we learned how ethnographers who harness LLMs as part of their toolkit are better equipped to surface new insights and connections to complement their work, increasing confidence in their qualitative methodologies. But only when the ethnographer applies their nuanced, contextual understanding of the user and organization to parse and iterate on what is and isn’t relevant.
The third experiment explored the extent to which LLMs can predict what travel videos are valued by users through an analysis of user generated comments. But it was only once ethnographers had decoded a complex space to teach the system what to “look for”, including complementary non-linguistic attributes, that those predictions became prescient.
LLMs are valuable to ethnographers, but they can’t intuit the dynamic social, cultural, political factors that shape value and meaning. In this sense they clarify the opportunity for the ethnographer to be the critical bridge between the “complex” worlds they explore and the “complicated” products and services that their work informs.
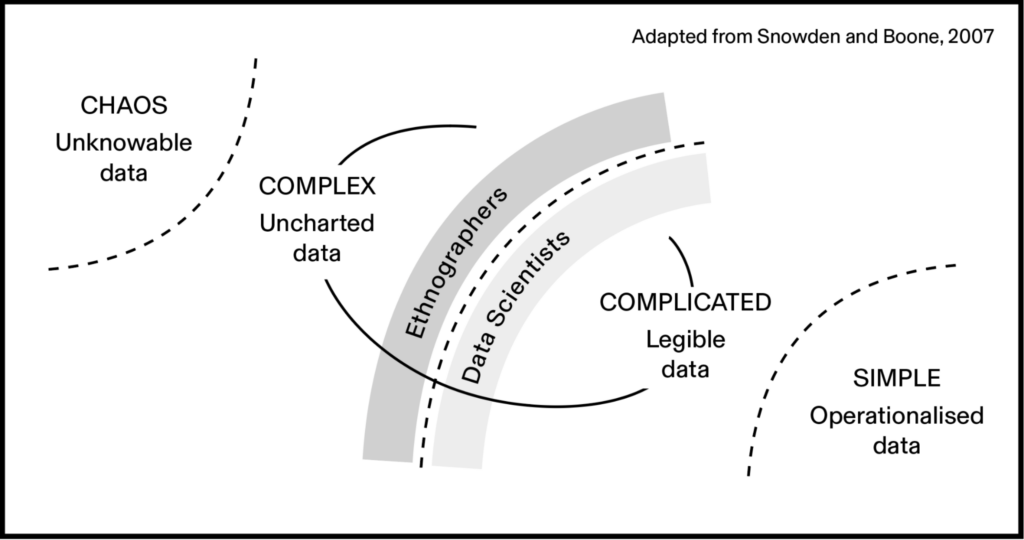
The increasing capacity of LLM’s has highlighted more precisely what researchers are uniquely capable of doing. As the relative value of ethnography is clarified, we foresee a new breed of data science-literate ethnographers emerging, who are able to work directly with LLMs (or collaborate more closely with data science colleagues on tasks that require more expert translation.)
In summary, ethnography can “ground” LLMs in two worlds: that of the user and that of the organisation. By understanding what is meaningful to users we can parse what kinds of clusters, classifications and searches are truly relevant. And by understanding what’s meaningful to organisations we can reframe and combine outputs to create greater impact.
LLMs have the capacity to help “scale” qualitative work, but not through an automated, closed loop platform. It is only when ethnographers and data scientists work closely together, skillfully adapting their tools in conversation with these worlds, that their true value is realized.
ABOUT THE AUTHORS
Tom Hoy is a founding Partner at Stripe Partners. He advises some of the world’s leading technology-led businesses on strategy and innovation. Over the last 18 months he has worked on the integration of Stripe Partners’ data science and social science teams, leading the development of new approaches to address novel client challenges. tom.hoy@stripepartners.com
Iman Munire Bilal is a part-time NLP researcher at Stripe Partners where she works to bring integrated quantitative-qualitative approaches to client projects. She is a final year PhD Candidate at Warwick University, UK. Her main interests lie in the field of NLP with applications in digital journalism and responsible AI. iman.bilal@stripepartners.com
Zoe Liou is a data scientist at Stripe Partners. She holds an MSc in Data Science from King’s College London, and a BSc in Economics from Soochow University. Her focus is on Natural Language Processing, particularly transforming rich textual data into actionable business contexts. zoe.liou@stripepartners.com
NOTES
Thank you to our clients for their collaboration and willingness to test new approaches across these experiments. Thank you to our paper curator Tiffany Romain for her advice and guidance throughout our paper editing process.
REFERENCES CITED
Amirebrahimi, S. 2016. The rise of the user and the fall of people: ethnographic cooptation and a new language of globalization. Ethnographic Praxis in Industry Conference Proceedings, 2016(1), 71-103. https://www.epicpeople.org/ethnographic-cooptation/
Anderson, K., Salvador, T., & Barnett, B. 2013.. Models in motion: Ethnography moves from complicatedness to complex systems. In Ethnographic Praxis in Industry Conference Proceedings (Vol. 2013, No. 1, pp. 232-249). https://www.epicpeople.org/models-in-motion-ethnography-moves-from-complicatedness-to-complex-systems/
Argyle, L. P., Busby, E. C., Fulda, N., Gubler, J. R., Rytting, C., & Wingate, D. 2022. Out of one, many: Using language models to simulate human samples. Political Analysis, 1-15.
Barbieri, F., Camacho-Collados, J., Espinosa Anke, L., & Neves, L. 2020. TweetEval: Unified benchmark and comparative evaluation for tweet classification. In Findings of the Association for Computational Linguistics: EMNLP 2020 (pp. 1644-1650). Online: Association for Computational Linguistics.
Beeferman, D., & Gillani, N. 2023. FeedbackMap: A tool for making sense of open-ended survey responses [Preprint]. arXiv. https://doi.org/10.48550/arXiv.2306.15112
Belt, S. 2019. Accelerating user research: How we structure insights for speed at Spotify. EPIC Perspectives. https://www.epicpeople.org/accelerating-user-research/
Bender, E., & Koller, A. 2020. Climbing towards NLU: On meaning, form, and understanding in the age of data. In Proceedings of the 58th Annual Meeting of the Association for Computational Linguistics (pp. 5185-5198). Online: Association for Computational Linguistics.
Bender, E. M., Gebru, T., McMillan-Major, A., & Shmitchell, S. 2021. On the dangers of stochastic parrots: Can language models be too big? In Proceedings of the 2021 ACM Conference on Fairness, Accountability, and Transparency (pp. 610-623).
Binz, M., & Schulz, E. 2023. Using cognitive psychology to understand GPT-3. In Proceedings of the National Academy of Sciences.
Brezulianu, A., Burlacu, A., Popa, I. V., Arif, M., & Geman, O. 2022. “Not by our feeling, but by other’s seeing”: Sentiment analysis technique in cardiology-An exploratory review. Frontiers in Public Health, 10, Article 880207. https://doi.org/10.3389/fpubh.2022.880207
Bowman, S. R. 2023. Eight things to know about large language models [Preprint]. arXiv. https://doi.org/10.48550/arXiv.2304.00612
Chomsky, N. 1975. Reflections on language. New York, NY: Pantheon.
Devlin, J., Chang, M.-W., Lee, K., & Toutanova, K. 2019. BERT: Pre-training of deep bidirectional transformers for language understanding. In Proceedings of the 2019 Conference of the North American Chapter of the Association for Computational Linguistics: Human Language Technologies, Volume 1 (Long and Short Papers) (pp. 4171-4186). Minneapolis, Minnesota: Association for Computational Linguistics.
Geertz, C. 2008. Thick description: Toward an interpretive theory of culture. In The cultural geography reader (pp. 41-51). Routledge.
Grootendorst, M. 2022. BERTopic: Neural topic modeling with a class-based TF-IDF procedure.
Harnad, S. 1990. The symbol grounding problem. Physica D: Nonlinear Phenomena, 42(1-3), 335-346.
Jacobin. 2021. Noam Chomsky: Militant optimism over climate crisis. https://jacobinmag.com/2021/03/noam-chomsky-climate-change-activism-optimism
Jentzsch, S., & Turan, C. 2022. Gender Bias in BERT – Measuring and Analysing Biases through Sentiment Rating in a Realistic Downstream Classification Task. In Proceedings of the 4th Workshop on Gender Bias in Natural Language Processing (GeBNLP) (pp. 184-199). Seattle, Washington: Association for Computational Linguistics.
Jones, P. 2015. Sensemaking methodology: A liberation theory of communicative agency. EPIC Perspectives. https://www.epicpeople.org/sensemaking-methodology/
Kaur, M., Costello, J., Willis, E., Kelm, K., Reformat, M. Z., & Bolduc, F. V. 2022. Deciphering the diversity of mental models in neurodevelopmental disorders: Knowledge graph representation of public data using natural language processing. Journal of Medical Internet Research, 24(8), Article e39888. https://doi.org/10.2196/39888
Lauretig, A. 2019. Identification, interpretability, and Bayesian word embeddings. In Proceedings of the Third Workshop on Natural Language Processing and Computational Social Science (pp. 7-17). Minneapolis, Minnesota: Association for Computational Linguistics.
Levin, N. 2019. Ethnographic agency in a data driven world. Ethnographic Praxis in Industry Conference Proceedings, 2019(1), 591-604. https://www.epicpeople.org/ethnographic-agency-data-driven-world/
Lucy, L., & Bamman, D. 2021. Gender and representation bias in GPT-3 generated stories. In Proceedings of the Third Workshop on Narrative Understanding (pp. 48-55). Virtual: Association for Computational Linguistics.
MacNamara, J. 2015. Sensemaking in organizations: Reflections on Karl Weick and social theory. EPIC Perspectives. https://www.epicpeople.org/sensemaking-in-organizations/
Meidinger, M., & Aßenmacher, M. 2021. A new benchmark for NLP in social sciences: Evaluating the usefulness of pre-trained language models for classifying open-ended survey responses.
Miotto, M., Rossberg, N., & Kleinberg, B. 2022. Who is GPT-3? An exploration of personality, values and demographics. In Proceedings of the Fifth Workshop on Natural Language Processing and Computational Social Science (NLP+CSS) (pp. 218-227). Abu Dhabi, UAE: Association for Computational Linguistics.
Nelson, L. K. 2020. Computational grounded theory: A methodological framework. Sociological Methods & Research, 49(1), 3-42. https://doi.org/10.1177/0049124117729703
Nelson, L. K. 2021. Leveraging the alignment between machine learning and intersectionality: Using word embeddings to measure intersectional experiences of the nineteenth century U.S. South. Poetics, 88, 101539. https://doi.org/10.1016/j.poetic.2021.101539
Park, A., & Conway, M. 2018. Tracking health related discussions on Reddit for public health applications. AMIA Annual Symposium Proceedings, 2017, 1362-1371. https://doi.org/29854205; PMCID: PMC5977623
Plantin, E. 1987. Mental models and metaphor. In Theoretical issues in natural language processing 3.
Roberts, S., & Hoy, T. 2015. Knowing that and knowing how: Towards Embodied Strategy. In Ethnographic Praxis in Industry Conference Proceedings (Vol. 2015, No. 1, pp. 306-321). https://www.epicpeople.org/knowing-that-and-knowing-how-towards-embodied-strategy/
Rogers, L. 2019. Bringing the security analyst into the loop: From human-computer interaction to human-computer collaboration. Ethnographic Praxis in Industry Conference Proceedings, 2019(1), 341-361.
Shen, C., Cheng, L., You, Y., & Bing, L. 2023. Are large language models good evaluators for abstractive summarization? [Preprint]. arXiv.
Snowden, D. J., & Boone, M. E. 2007. A leader’s framework for decision making. Harvard business review, 85(11), 68.
Stevenson, C., Smal, I., Baas, M., Grasman, R., & Maas, H. 2022. Putting GPT-3’s creativity to the (Alternative Uses) Test [Preprint]. arXiv. https://doi.org/10.48550/arXiv.2206.08932
Tedeschi, S., Bos, J., Declerck, T., Hajic, J., Hershcovich, D., Hovy, E. H., Koller, A., Krek, S., Schockaert, S., Sennrich, R., Shutova, E., & Navigli, R. 2023. What’s the meaning of superhuman performance in today’s NLU? Association for Computational Linguistics.
The Critique. 2022. Chomsky to Butterfield: Don’t parrot State and Power. https://thecritique.com/interviews/chomsky-to-butterfield-dont-parrot-state-and-power/
Touileb, S., & Nozza, D. 2022. Measuring harmful representations in Scandinavian language models. In Proceedings of the Fifth Workshop on Natural Language Processing and Computational Social Science (NLP+CSS) (pp. 118-125). Abu Dhabi, UAE: Association for Computational Linguistics.
Weidinger, L., Mellor, J., Rauh, M., Griffin, C., Uesato, J., Huang, P. S., Cheng, M., Glaese, M., Balle, B., Kasirzadeh, A., & Kenton, Z. 2021. Ethical and social risks of harm from language models [Preprint]. arXiv. https://doi.org/10.48550/arXiv.2112.04359
Weick, K. 1995. Sensemaking in organizations.
Wei, J., Wang, X., Schuurmans, D., Bosma, M., Ichter, B., Xia, F., Chi, E.H., Le, Q.V., & Zhou, D. 2023. Chain-of-thought prompting elicits reasoning in large language models.
Xu, X., Stulp, G., Van Den Bosch, A., & Gauthier, A. 2022. Understanding narratives from demographic survey data: A comparative study with multiple neural topic models. In Proceedings of the Fifth Workshop on Natural Language Processing and Computational Social Science (NLP+CSS) (pp. 33-38). Abu Dhabi, UAE: Association for Computational Linguistics.
Yadav, S., Ekbal, A., Saha, S., & Bhattacharyya, P. 2018. Medical sentiment analysis using social media: Towards building a patient assisted system. In Proceedings of the Eleventh International Conference on Language Resources and Evaluation (LREC 2018). Miyazaki, Japan: European Language Resources Association (ELRA).